Content from Запуск та завершення роботи
Останнє оновлення 2025-04-24 | Редагувати цю сторінку
Огляд
Питання
- Як запустити програми Python?
Цілі
- Запуск серверу JupyterLab.
- Створення нового скрипту Python.
- Створення блокноту Jupyter.
- Завершення роботи сервера JupyterLab.
- Розуміння різниці між скриптом Python і блокнотом Jupyter.
- Створення в блокноті комірок типу Markdown.
- Створення та виконання в блокноті комірок Python.
Для роботи з Python, протягом цього семінару ми будемо використовувати [блокноти Jupyter][jupyter] у середовищі JupyterLab. Блокноти Jupyter широко застосовуються з метою аналізу та візуалізації даних, а також є зручним інструментальним засобом для запуску коду на Python в інтерактивному режимі, де ми можемо легко переглядати результати його виконання, та ділитися нашим кодом з іншими.
Існують й інші способи редагування, організації та виконання коду. Розробники програмного забезпечення часто використовують інтегроване середовище розробки (IDE), подібне до PyCharm або Visual Studio Code або текстові редактори такі як Vim або Emacs, щоб створити та відредагувати свої програми Python. Після редагування та збереження ваших програм Python ви можете виконувати ці програми в самому IDE або безпосередньо в командному рядку. На відміну від цього, блокноти Jupyter дозволяють відразу переглянути результати нашого Python коду.
JupyterLab має декілька інших зручних функцій:
- Ви можете легко вводити, редагувати, копіювати та вставляти блоки коду.
- Автодоповнення за допомогою клавіші Tab дозволяє легко отримувати доступ до назв об’єктів, які ви використовуєте.
- Дозволяє легко доповнювати свій код посиланнями, текстом різного розміру, маркерами тощо, щоб зробити його доступнішим для вас і ваших колег.
- Дозволяє розміщувати графічні елементи безпосередньо поруч із кодом, який їх створює, щоб продемонструвати повну історію аналізу даних.
Кожен блокнот містить одну або кілька комірок, що містять код, текст або зображення.
Початок роботи з JupyterLab
JupyterLab є сервером застосунків із вебінтерфейсом користувача від Project Jupyter, що дозволяє працювати з документами та іншими застосунками, такими як блокноти Jupyter, текстові редактори, термінали, і навіть спеціальні компоненти, гнучким, інтегрованим і розширюваним способом. JupyterLab потребує досить сучасний браузер (в ідеалі – поточна версія Chrome, Safari або Firefox); Internet Explorer версії 9 і нижче не підтримується.
JupyterLab є частиною інсталяційного пакета Anaconda Python. Якщо ви не встановили дистрибутив Anaconda Python, дивіться інструкції щодо процесу інсталяції тут.
На цьому уроці ми запустимо JupyterLab локально на наших власних пристроях, тому для цього підключення до Інтернету буде потрібно лише на початку для завантаження та встановлення середовищ розробки Anaconda та JupyterLab
- Запустіть сервер JupyterLab на вашому комп’ютері
- Використовуйте веббраузер для відкриття спеціальної локальної URL-адреси для з’єднання з сервером JupyterLab
- Сервер JupyterLab виконує обчислювальну роботу, а веббраузер відображає її результат
- Введіть код у браузері, і як тільки сервер JupyterLab завершить виконання, ви зможете переглянути результати
JupyterLab? А чому не Jupyter Notebook?
JupyterLab є подальшим кроком в еволюції Jupyter Notebook. Якщо ви використовували Jupyter Notebook раніше, то ви добре зрозумієте діапазон можливостей JupyterLab.
Досвідчені користувачі блокнотів Jupyter, зацікавлені у більш детальному обговоренні схожостей і відмінностей між інтерфейсами JupyterLab і Jupyter Notebook, можуть знайти більше інформації у документації з інтерфейсу користувача JupyterLab.
Початок роботи з JupyterLab
Ви можете запустити сервер JupyterLab через командний рядок або через застосунок, що має назву ‘Anaconda Navigator’. JupyterLab є частиною інсталяційного пакета Anaconda Python.
macOS - командний рядок
Для запуску сервера JupyterLab вам потрібно отримати доступ до командного рядка через Terminal. Існує два способи відкрити термінал на Mac.
- У каталозі Applications відкрийте підкаталог Utilities і двічі натисніть Terminal
- Натисніть Command + spacebar для запуску
Spotlight. Введіть
Terminal
, а потім двічі клацніть на результаті пошуку або натисніть Enter
Після запуску Terminal введіть команду для запуску сервера JupyterLab.
Користувачі Windows - Командний рядок
Для запуску сервера JupyterLab вам потрібен застосунок Anaconda Prompt.
Натисніть Windows Logo Key і знайдіть
Anaconda Prompt
, натисніть на результат пошуку або на
клавішу enter.
Після запуску Anaconda Prompt введіть команду:
Anaconda Navigator
Для запуску серверу JupyterLab з Anaconda Navigator ви маєте спочатку
запустити
Anaconda Navigator (натисніть для докладних інструкцій з macOS, Windows
та Linux). Ви можете виконати пошук Anaconda Navigator через
Spotlight на macOS (Command + spacebar),
скористатися функцією пошуку Windows (ключ Windows Logo) або
відкривши термінал та виконавши команду anaconda-navigator
у командному рядку.
Після того, як ви запустили Anaconda Navigator, натисніть кнопку
Launch
під JupyterLab. Можливо, вам знадобиться продивитись
список донизу, аби знайти її.
Нижче наведено скриншот сторінки Anaconda Navigator, схожої на ту, яка має відкриватися для macOS або Windows.
Нижче наведено скриншот екрана стартової сторінки JupyterLab, схожої на ту, яка має відкритися у вашому веббраузері за замовчуванням після запуску сервера JupyterLab в операційній системі macOS або Windows.
Інтерфейс JupyterLab
JupyterLab має багато функцій, які можна знайти в традиційних інтегрованих середовищах розробки (IDE), але його особливістю є забезпечення гнучких “будівельних блоків” для інтерактивних дослідницьких обчислень.
Інтерфейс JupyterLab складається з панелі меню, лівої бічної панелі (що згортається за потреби), і основної робочої області, яка містить вкладки з документами та різними застосунками JupyterLab.
Панель меню
Панель меню у верхній частині вікна JupyterLab містить меню верхнього рівня, яке зображує різні дії доступні в JupyterLab разом із їхніми комбінаціями клавіш (де це можливо). Наступні пункти меню наявні за замовчуванням.
- File: Дії, пов’язані з файлами та каталогами, такі як New, Open, Close, Save тощо. Меню File також містить дію Shut Down, яка застосовується для завершення роботи сервера JupyterLab.
- Edit: Дії, пов’язані з редагуванням документів та іншими видами діяльності, такими як Undo, Cut, Copy, Paste тощо.
- View: Дії, які змінюють зовнішній вигляд інтерфейсу JupyterLab.
- Run: Дії для запуску коду в різних застосунках, таких як Jupyter Notebook та командний рядок (розглянуто нижче).
- ** Kernel:** Дії щодо управління ядрами. Ядра у Jupyter будуть детально описані нижче.
- Tabs: Список відкритих документів та застосунків у робочій області.
- Settings: За допомогою цього меню можна налаштувати загальні параметри JupyterLab. Окрім того, у ньому також є опція Advanced Settings Editor, яка забезпечує більш детальний контроль параметрів і опцій для конфігурації JupyterLab.
- Help: Список посилань на довідку JupyterLab та інші ресурси.
Ядра
Документація JupyterLab визначає ядра як “окремі процеси сервера, що виконують ваш код у відповідних мовах програмування та середовищах.” Коли ми відкриваємо Jupyter Notebook, то ініціалізується ядро - процес, який буде виконувати код. У цьому уроці ми будемо використовувати ядро ipython, яке дозволяє запускати Python 3 в інтерактивному режимі.
Використання інших ядер Jupyter для інших мов програмування дозволить нам використовувати спільний інтерфейс JupyterLab для того, щоб писати та виконувати код, наприклад, у таких мовах як R, Java, Julia, Ruby, JavaScript, Fortran, тощо.
Скриншот стандартної панелі меню надано нижче.
Ліва бічна панель
Ліва бічна панель містить ряд найбільш використовуваних вкладок, а саме: браузер файлів (відображає вміст каталогу, де був запущений сервер JupyterLab), перелік активних ядер і терміналів, панель команд і список відкритих вкладок в основній робочій області. Скриншот стандартної лівої бічної панелі наведений нижче.
Ліву бічну панель можна згорнути або розгорнути вибравши пункт “Show Left Sidebar” у меню View, або натиснувши на активну вкладку бічної панелі.
Основна робоча область
Основна робоча область в JupyterLab дозволяє упорядковувати документи (блокноти, текстові файли та ін.) та інші види застосунків (термінали, інтерфейси командного рядка тощо) у панелі вкладок. Ці панелі можна зменшити/збільшити або поділити на підрозділи. Скриншот стандартної основної робочої області наведено нижче.
Якщо Ви не бачите вкладку Launcher на панелі запуску, натисніть синій плюс під “File” та “Edit” у панелі меню, і ця вкладка з’явиться.
Щоб перемістити вкладку на деяку панель, перетягніть її в центр цієї панелі. Також ви можете розділити панель вкладок, перетягнувши потрібну вкладку ліворуч, праворуч, догори або донизу панелі. Кожна робоча панель має одну поточну активну вкладку. Вкладка для поточної дії позначена кольоровою верхньою рамкою (за замовчуванням - синьою).
Створення скрипту Python
- Щоб почати писати нову програму на Python, натисніть піктограму
текстового файлу під заголовком Other на вкладці Launcher
(Запуск) головної робочої області.
- Можна також створити новий текстовий файл, якщо обрати New -> Text File у меню File на панелі меню.
- Щоб перетворити цей звичайний текстовий файл на програму Python,
виберіть дію Save File As у меню File на панелі меню
та надайте новому текстовому файлу назву, яка закінчується розширенням
.py
.- Розширення
.py
повідомляє всім (операційній системі включно), що цей текстовий файл є програмою Python. - Це умовність, а не вимога.
- Розширення
Створення блокноту Jupyter
Щоб відкрити новий блокнот, натисніть піктограму Python 3 під заголовком Notebook на вкладці Launcher в у головній робочій області. Ви також можете створити новий блокнот, обравши New -> Notebook у меню File на панелі меню.
Додаткові зауваження щодо блокнотів Jupyter.
- Файли, створені в Jupyter Notebook, мають розширення
.ipynb
, щоб відрізнити їх від програм на Python, створених як звичайний текстовий файл. - Блокноти можна експортувати як скрипти Python, які можна запускати з командного рядка.
Нижче наведено скриншот Jupyter Notebook, який був відкритий в JupyterLab. Якщо вам цікаві подальші деталі, дивіться офіційну документацію до Jupyter Notebook.
Як це зберігається
- Файл блокноту зберігається у форматі JSON.
- Подібно до вебсторінки, те, що зберігається, відрізняється від того, що ви бачите у своєму браузері.
- Але формат JSON дозволяє Jupyter комбінувати вихідний код, текст і графіку в одному файлі.
Упорядкування документів в панелях вкладок
У головній робочій області JupyterLab ви можете впорядковувати документи на панелі вкладок. Нижче наведено приклад з офіційної документації.
Спочатку створіть текстовий файл, консоль Python, та вікно терміналу і розташуйте їх у три панелі в основній робочій області. Далі створіть блокнот, вікно терміналу, та текстовий файл і розподіліть їх на три панелі в основній робочій зоні. Нарешті, створіть власну комбінацію панелей і вкладок. Яка, на вашу думку, комбінація панелей та вкладок буде найбільш корисною для вашого робочого процесу?
Після створення необхідних вкладок ви можете перетягнути одну з них в центр панелі для переміщення вкладки на панель; потім ви можете розділити панель, перетягнувши вкладку ліворуч, праворуч, вгору або до низу панелі.
Код або текст?
Jupyter дозволяє змішувати код і текст у різних типах блоків, які називаються комірками. Термін “код” зазвичай використовується для позначення вихідного коду програмного забезпечення, написаного будь-якою мовою програмування. “Комірка коду” в Notebook містить код; а “текстова комірка” - звичайний текст, який відображується, але не виконується.
Jupyter Notebook має командний режим та режим редагування.
- Якщо ви натиснете Esc та Return по черзі, то зовнішня межа комірки коду буде змінюватися з сірої на синю.
- Існують сірий - Command (командний) та синій - Edit (редагування) режими вашого блокноту.
- Command mode allows you to edit notebook-level features, and Edit mode changes the content of cells.
- В командному режимі (esc/сірий),
- Клавіша b створює нову комірку нижче поточної обраної комірки.
- Клавіша a створює одну комірку вище поточної.
- Клавіша x видаляє поточну комірку.
- Клавіша z скасовує вашу останню операцію з коміркою (це може бути операція видалення, створення тощо).
- Усі дії можна виконувати за допомогою меню, але є багато комбінацій клавіш для прискорення процесу.
Командний режим або режим редагування
Чи ви зараз перебуваєте в командному режимі чи режимі редагування на
сторінці Jupyter Notebook?
Перейдіть з одного режиму в інший, а потім у зворотному напрямку.
Використайте відповідні швидкі клавіші для видалення комірки.
Використайте швидкі клавіші, щоб видалити комірку. Нарешті, скасуйте
останню операцію над коміркою також за допомогою швидких клавіш.
Командний режим має сіру рамку, а режим редагування — синю. Використовуйте Esc та Return для перемикання режимів. Ви маєте бути в командному режимі (Натисніть Esc якщо ваша комірка синя). Введіть b або a. Ви маєте бути в командному режимі (Натисніть Esc якщо ваша клітинка синя). Введіть x. Ви маєте бути в командному режимі (Натисніть Esc якщо ваша комірка синя). Введіть z.
Використовуйте клавіатуру та мишу для виділення та редагування комірок.
- Якщо натиснути клавішу Return, рамка стане синьою та ввімкнеться режим редагування, що дозволяє введення команди в комірку.
- Якщо є необхідність введення кількох рядків кода в одну клітинку, то натискання клавіші Return в режимі редагування (синя рамка) переміщує курсор на наступний рядок в комірці, як у текстовому редакторі.
- Якщо нам потрібно запустити код, що знаходиться в комірці, нам потрібен інший спосіб повідомити про це Notebook.
- Одночасне натискання клавіш Shift + Return призведе до виконання вмісту комірки.
- Зверніть увагу, що клавіші Return та Shift розташовані поруч на клавіатурі справа.
Jupyter Notebook підтримує мову розмітки текстів Markdown.
- Блокноти також можуть візуалізувати Markdown.
- Простий текстовий формат для створення списків, посилань та інших елементів, які можуть бути використані на вебсторінці.
- Власне, це підмножина HTML, яка виглядає у стилі старомодного електронного листа.
- Перетворіть поточну комірку на комірку Markdown, увійшовши в командний режим (Esc/gray) та натиснувши клавішу M.
- Позначка
In [ ]:
зникне, щоб показати, що це вже не комірка коду, і ви зможете писати текст у форматі Markdown. - Перетворіть поточну комірку на комірку з кодом, увійшовши в командний режим (Esc/gray) та натиснувши клавішу y.
Markdown робить більшість того, що можна зробити у HTML.
Таблиця: Елементи синтаксису Markdown та їх зображення.
+—————————————+————————————————+ | Markdown code | Rendered output |
+=======================================+================================================+
+—————————————+————————————————+ |
| <p></p> | | * Use asterisks | - Use asterisks | | * to create | - to create | | * bullet lists. | - bullet lists. | |
| | +—————————————+————————————————+ +—————————————+————————————————+ |
| <p></p> | | 1. Use numbers | 1. Use numbers | | 1. to create | 2. to create | | 1. bullet lists. | 3. numbered lists. | |
| | +—————————————+————————————————+ +—————————————+————————————————+ |
| <p></p> | | * You can use indents | - You can use indents | | * To create sublists | - To create sublists | | * of the same type | - of the same type | | * Or sublists | - Or sublists | | 1. Of different | 1. Of different | | 1. types | 2. types | |
| | +—————————————+————————————————+ +—————————————+————————————————+ |
| <p></p> | | # A Level-1 Heading | ## A Level-1 Heading | |
| | +—————————————+————————————————+ +—————————————+————————————————+ |
| <p></p> | | ## A Level-2 Heading (etc.) | ### A Level-2 Heading (etc.) | |
| | +—————————————+————————————————+ +—————————————+————————————————+ |
| <p></p> | | Line breaks | Line breaks | | don't matter. | don't matter. | | | | | But blank lines | But blank lines | | create new paragraphs. | create new paragraphs. | |
| | +—————————————+————————————————+ +—————————————+————————————————+ |
| <p></p> | | [Links](http://software-carpentry.org)| [Links](https://software-carpentry.org) | | are created with `[...](...)`. | are created with `[...](...)`. | | Or use [named links][data-carp]. | Or use [named links][data_carpentry]. | | | | | [data-carp]: http://datacarpentry.org | | |
| | +—————————————+————————————————+
Створення списків в Markdown
Створіть вкладений список в Markdown-комірці блокноту так, щоб вона виглядала наступним чином:
- Знайти фінансування.
- Виконати роботу.
- Провести експеримент.
- Зібрати дані.
- Провести аналіз.
- Написати статтю.
- Опублікувати.
Це завдання поєднує як нумерований, так і маркований списки. Зверніть увагу, що маркований список має відступ на 2 пробіли, щоб він не збігався з елементами нумерованого списку.
1. Get funding.
2. Do work.
* Design experiment.
* Collect data.
* Analyze.
3. Write up.
4. Publish.
Зміна типу вже наявної комірки з Code на Markdown
Що станеться, якщо Ви напишете у комірці код Python, а потім перемкнете її у режим Markdown? Наприклад, напишіть наступний вираз в комірці коду:
Потім запустіть цей код в комірці за допомогою Shift+Return, щоб переконатися, що ця комірка працює як комірка коду. Тепер поверніться до комірки та натисніть Esc, а потім m, щоб перемкнути комірку на Markdown і “запустити” її за допомогою Shift+Return. Що сталося, і як це може бути корисним?
Рівняння
Стандартний Markdown (наприклад, такий, що використовується для цих нотаток) не відображає рівняння, але Notebook буде це робити. Створіть нову комірку Markdown і введіть наступне:
$\sum_{i=1}^{N} 2^{-i} \approx 1$
(Мабуть, це легше скопіювати та вставити.) Що зображається? Як ви
думаєте, що роблять підкреслювання _
, циркумфлекс
^
і знак долара $
?
Рівняння зображується у блокноті відповідно до синтаксису, який
використовується у LaTeX. Знаки долара $
використовуються
для того, щоб повідомити Markdown про те, що текст між цими знаками є
рівнянням LaTeX. Якщо ви не знайомі з LaTeX, підкреслення _
використовується для підрядкових індексів та циркумфлекс ^
використовується для верхніх індексів. Пара фігурних дужок
{
та }
використовується для групування тексту
разом, щоб вираз i=1
став нижнім, а N
-
верхнім індексом. Аналогічно, вираз -i
взятий у фігурні
дужки, щоб зробити цей вираз верхнім індексом для 2
.
\sum
та \approx
є командами LaTeX для значень
“sum over” й “approximate”.
Вихід з JupyterLab
- На панелі меню оберіть меню “File” і натисніть “Shut Down” внизу спадного меню. Вам буде запропоновано підтвердити, що Ви бажаєте вимкнути сервер JupyterLab (не забудьте зберегти свою роботу!). Натисніть “Shut Down”, щоб вимкнути сервер JupyterLab.
- Щоб перезапустити сервер JupyterLab, вам потрібно буде повторно виконати наступну команду в терміналі.
$ jupyter lab
Вихід з JupyterLab
Потренуйтеся закривати та перезапускати сервер JupyterLab.
Ключові моменти
- Скрипти Python - це звичайні текстові файли.
- Застосування Jupyter Notebook для редагування та запуску Python
- Jupyter Notebook має командний режим та режим редагування.
- Використовуйте клавіатуру та мишу для виділення та редагування комірок.
- Notebook підтримує мову розмітки текстів Markdown.
- Markdown робить більшість того, що можна зробити у HTML.
Content from Змінні та присвоєння
Останнє оновлення 2025-04-03 | Редагувати цю сторінку
Огляд
Питання
- Як я можу зберігати дані в програмах?
Цілі
- Створення програм, які присвоюють скалярні значення змінним і виконують обчислення з цими значеннями.
- Відстеження у програмах значень змінних, які використовують скалярне присвоєння.
Використовуйте змінні для зберігання значень.
Змінні - це імена значень.
-
Імена змінних
- можуть складатися тільки з букв, цифр та
підкреслення
_
(яке звичайно використовується, щоб відокремити слова у довгих назвах змінних) - не можуть починатися з цифри
-
залежать від регістру (тобто
age
,Age
таAGE
- це три різні змінні)
- можуть складатися тільки з букв, цифр та
підкреслення
Ім’я змінної має бути змістовним, щоб ви або інший програміст знали, що це таке
Імена змінних, які починаються з підкреслення, наприклад
__alistairs_real_age
, мають специфічне значення, тому ми не будемо цього робити, доки не зрозуміємо прийняті в мові Python домовленості.У Python символ
=
використовується для присвоєння значення, яке знаходиться праворуч, до його імені, яке вказано ліворуч.Змінна створена, коли їй присвоюється значення.
-
У виразі нижче Python призначає вік змінній
age
та ім’я в лапках - зміннійfirst_name
.
Використовуйте print
для виведення значень.
- Python має вбудовану функцію
print
, яка друкує щось як текст. - Щоб викликати функцію (тобто, щоб виконати її), треба вказати її ім’я.
- Щоб передати функції значення (тобто дані для друку), їх треба помістити у дужки.
- Щоб направити до друку рядок тексту, його треба помістити в одинарні або подвійні лапки.
- Значення, які передаються до функції, називаються аргументами
ВИХІД
Ahmed is 42 years old
-
print
автоматично додає пробіл між надрукованими аргументами, щоб відокремити їх. - Також
print
переходить на новий рядок після друку.
Змінні мають бути створені перед їх використанням.
- Якщо змінна ще не існує, або якщо ім’я було неправильно написано, Python повідомляє про помилку. (На відміну від деяких інших мов, які можуть “вгадати” якесь значення за замовчуванням.)
ПОМИЛКА
---------------------------------------------------------------------------
NameError Traceback (most recent call last)
<ipython-input-1-c1fbb4e96102> in <module>()
----> 1 print(last_name)
NameError: name 'last_name' is not defined
- Останній рядок у повідомленні про помилку є найбільш інформативним.
- Ми більш детально розглянемо повідомлення про помилки пізніше.
Змінні зберігаються між комірками
Майте на увазі, що в блокноті Jupyter важливий порядок виконання комірок, а не порядок їх розташування. Python запам’ятає весь код, який було виконано раніше, у тому числі всі змінні, які ви визначили, незалежно від порядку в блокноті. Тому, якщо ви визначите змінні нижче в блокноті, а потім (повторно) запустите комірки вище, то ті комірки, що визначені нижче, все одно будуть присутні. Як приклад, створіть дві комірки наступного вмісту у такому порядку:
Якщо виконати це послідовно, то перша комірка дасть помилку. Однак,
якщо ви запустите першу комірку після другої, вона виведе на
екран 1
. Щоб уникнути плутанини, можна скористатися опцією
Kernel
-> Restart & Run All
, яка
перезавантажує інтерпретатор і запускає все з чистого аркуша, зверху
вниз.
Змінні можна використовувати для обчислень.
- Ми можемо використовувати змінні в обчисленнях так само, як би вони
були значеннями.
- Пам’ятайте, ми присвоїли значення
42
зміннійage
кількома рядками вище.
- Пам’ятайте, ми присвоїли значення
ВИХІД
Age in three years: 45
Використовуйте індекс, щоб отримати один символ із рядка.
- Символи (окремі літери, цифри тощо) у рядку є впорядкованими.
Наприклад, рядок
'AB'
не те саме, що'BA'
. Завдяки такому упорядкуванню ми можемо розглядати рядок як список символів. - Кожна позиція в рядку (перша, друга тощо) має номер. Це число називається індексом або іноді нижнім індексом.
- Індекси нумеруються від 0.
- Використовуйте індекс позиції у квадратних дужках, щоб отримати символ з тієї позиції у рядку.
ВИХІД
h
Використовуйте зріз, щоб отримати підрядок.
- Частина рядка називається підрядок. Він може складатися навіть тільки з одного символу.
- Список складається з елементів. У випадку, коли рядок розглядається як список, його елементами є окремі символи.
- Зріз - це частина рядка (в загальному випадку, частина будь-якого обʼєкту, схожого на колекцію).
- Ми беремо зріз із позначенням
[start:stop]
, деstart
— ціле число, що є індексом першого потрібного нам елементу, аstop
- ціле число, що є індексом елементу відразу після останнього потрібного нам елементу. - Проміжок між
stop
andstart
- це довжина зрізу. - Визначення зрізу не змінює вміст вихідного рядка. Натомість, визначений зріз повертає копію початкового рядка.
ВИХІД
sod
Використовуйте вбудовану функцію len
, щоб знайти
довжину рядка.
ВИХІД
6
- Вкладені функції обчислюються зсередини назовні, як у математиці: вирази, що знаходяться всередині дужок, обчислюються першими.
Python чутливий до регістру.
- Python вважає, що букви верхнього та нижнього регістру
відрізняються, отже
Name
іname
- різні змінні. - Існують домовленості про використання великих літер на початку імен змінних, тому ми будемо використовувати малі літери.
Використовуйте змістовні назви змінних.
- Ви можете використовувати будь-які комбінації символів для імен змінних, поки вони задовольняють вищевказані правила (букви, цифри та знак підкреслення).
- Використовуйте змістовні назви змінних, щоб допомогти іншим зрозуміти, що робить програма.
- Найважливіша “інша людина” — це ви в майбутньому.
ВИХІД
# Оператор # Значення x # Значення y # Значення swap #
x = 1.0 # 1.0 # не визначено # не визначено #
y = 3.0 # 1.0 # 3.0 # не визначено #
swap = x # 1.0 # 3.0 # 1.0 #
x = y # 3.0 # 3.0 # 1.0 #
y = swap # 3.0 # 1.0 # 1.0 #
Ці три рядки обмінюються значеннями в x
і y
використовуючи змінну swap
для тимчасового зберігання. Це
досить поширена ідіома програмування.
Завдання
Якщо ви присвоїли a = 123
, що станеться, якщо ви
спробуєте отримати другу цифру a
через
a[1]
?
Числа не є рядками або послідовностями, і спроба виконати операцію
індексу над числом у Python призведе до помилки. У наступному епізоді про типи даних і
перетворення типів ми дізнаємось більше про типи і як конвертувати
один тип в інший. Якщо вам потрібна N-та цифра числа, перетворіть його
на рядок за допомогою вбудованої функції str
, а потім
виконайте операцію індексації у цьому рядку.
ПОМИЛКА
TypeError: 'int' object is not subscriptable
ВИХІД
2
Вибір імені
Яке ім’я для змінної є кращим: m
, min
або
minutes
? Чому? Підказка: подумайте, який код ви б хотіли
успадкувати від того, хто залишає лабораторію:
ts = m * 60 + s
tot_sec = min * 60 + sec
total_seconds = minutes * 60 + seconds
minutes
краще, оскільки min
можна помилково
прийняти за “мінімум” (що насправді є вбудованою функцією в Python, яку
ми розглянемо пізніше).
ВИХІД
atom_name[1:3] is: ar
Різноманітні види зрізів
Є наступний рядок:
Що виводять ці вирази?
species_name[2:8]
-
species_name[11:]
(без значення після двокрапки) -
species_name[:4]
(без значення до двокрапки) -
species_name[:]
(тільки двокрапка) species_name[11:-3]
species_name[-5:-3]
- Що станеться, якщо ми оберемо значення
stop
, яке виходить за рамки діапазону? (тобто спробуйте виконатиspecies_name[0:20]
абоspecies_name[:103]
)
species_name[2:8]
повертає підрядок'acia b'
species_name[11:]
повертає підрядок'folia'
, з позиції 11 до кінця рядкуspecies_name[:4]
повертає підрядокAcac'
, з початку рядку до позиції 4, не включаючи цю позиціюspecies_name[:]
повертає весь рядок ‘Acacia buxifolia’`species_name[11:-3]
повертає підрядок'fo'
, з 11 позиції до третьої позиції з кінця рядку, не включаючи їїspecies_name[-5:-3]
також повертає підрядок'fo'
, з п’ятої позиції з кінця до третьої позиції з кінця, не включаючи їїЯкщо частина фрагмента виходить за межі діапазону, операція не повертає помилку.
species_name[0:20]
дає той самий результат, що іspecies_name[0:]
, таspecies_name[:103]
дає такий самий результат, якspecies_name[:]
Ключові моменти
- Використовуйте змінні для зберігання значень.
- Використовуйте
print
для виводу значень. - Змінні зберігаються між комірками.
- Змінні мають бути створені перед їх використанням.
- Змінні можна використовувати для обчислень.
- Використовуйте індекс, щоб отримати один символ із рядка.
- Використовуйте зріз, щоб отримати підрядок.
- Використовуйте вбудовану функцію
len
, щоб знайти довжину рядка. - У Python важливо, який регістр використовується.
- Використовуйте змістовні назви змінних.
Content from Типи даних та їх перетворення
Останнє оновлення 2025-04-03 | Редагувати цю сторінку
Огляд
Питання
- Які види даних зберігають програми?
- Як я можу перетворити один тип в інший?
Цілі
- Визначення ключових відмінностей між цілими числами та числами з плаваючою комою.
- З’ясувати ключові відмінності між числами та символьними рядками.
- Використання вбудованих функцій для перетворення цілих чисел, чисел з плаваючою комою та рядків.
Кожне значення має тип.
- Кожне значення, яке використовує програма, має певний тип.
- Ціле число (
int
): зображує додатні або від’ємні цілі числа, наприклад 3 або -512. - Число з плаваючою комою (
float
): зображує дійсні числа, наприклад 3.14159 або -2.5. - Рядки символів (зазвичай просто “рядки”,
str
): представляють текст.- Укладені в одинарні або подвійні лапки (тип лапок має збігатися).
- Під час відображення рядку лапки не друкуються.
Вбудована функція type
повертає тип значення.
- Використовуйте вбудовану функцію
type
, щоб з’ясувати, який тип має значення. - Це також працює зі змінними.
- Але запамʼятайте: значення має свій тип, а змінна тільки вказує на деяке значення.
ВИХІД
<class 'int'>
ВИХІД
<class 'str'>
Тип визначає, які операції (або методи) можна виконувати над даним значенням.
- Тип значення визначає, що може робити з ним програма.
ВИХІД
2
ПОМИЛКА
---------------------------------------------------------------------------
TypeError Traceback (most recent call last)
<ipython-input-2-67f5626a1e07> in <module>()
----> 1 print('hello' - 'h')
TypeError: unsupported operand type(s) for -: 'str' and 'str'
Ви можете використовувати оператори “+” та “*” для дій над рядками.
- “Додавання” рядків об’єднує їх.
ВИХІД
Ahmed Walsh
- Якщо рядок помножити на ціле число N, то це створить новий
рядок, який буде містити вихідний рядок, повторений N разів.
- Оскільки множення - це повторюване додавання.
ВИХІД
==========
Рядки мають довжину (але числа її не мають).
- Вбудована функція
len
повертає кількість символів у рядку.
ВИХІД
11
- Але числа не мають довжини (навіть нульової).
ПОМИЛКА
---------------------------------------------------------------------------
TypeError Traceback (most recent call last)
<ipython-input-3-f769e8e8097d> in <module>()
----> 1 print(len(52))
TypeError: object of type 'int' has no len()
Необхідно перетворювати числа в рядки або навпаки під час виконання певних операцій.
- Додавання чисел та рядків неможливе.
ПОМИЛКА
---------------------------------------------------------------------------
TypeError Traceback (most recent call last)
<ipython-input-4-fe4f54a023c6> in <module>()
----> 1 print(1 + '2')
TypeError: unsupported operand type(s) for +: 'int' and 'str'
- Таке додавання не дозволено, тому що воно не визначене: чи має
1 + '2'
повертати3
чи'12'
? - Перетворення типу виконується за допомогою функції, яка має те ж саме імʼя, що і потрібний тип.
ВИХІД
3
12
З цілими та дійсними числами можна оперувати одночасно.
- Цілі та дійсні числа можна використовувати разом для арифметичних
дій.
- Python 3 автоматично перетворить цілі числа у дійсні, якщо це потрібно.
ВИХІД
half is 0.5
three squared is 9.0
Змінні можуть змінити своє значення тільки через присвоєння.
- Якщо в електронних таблицях ми зробимо одну комірку залежною від іншої та оновимо останню, перша оновиться автоматично.
- Це не трапляється у мовах програмування.
PYTHON
variable_one = 1
variable_two = 5 * variable_one
variable_one = 2
print('first is', variable_one, 'and second is', variable_two)
ВИХІД
first is 2 and second is 5
- Комп’ютер зчитує значення
variable_one
під час множення, створює нове значення та призначає цьому значенню ім’яvariable_two
. - Після того, як значення
variable_two
встановлено, воно не залежить від значенняvariable_one
, отже його значення не змінюється автоматично, колиvariable_one
змінюється.
Дроби
Який тип має значення 3.4? Як це можна встановити?
Автоматичне перетворення типів
Який тип має вираз 3.25 + 4?
Вибір типу
Який тип значення (ціле число, число з плаваючою комою або рядок символів) ви б використовували для представлення кожного з наступних значень? Спробуйте знайти більш ніж одну гарну відповідь для кожної проблеми. Наприклад у завданні # 1, коли було б доцільніше використовувати змінну з плаваючою комою замість цілого числа для підрахунку днів?
- Кількість днів, які пройшли з початку року.
- Час, що пройшов від початку року до сьогоднішнього дня.
- Серійний номер лабораторного обладнання.
- Вік лабораторного зразка
- Поточне населення міста.
- Середня чисельність населення міста протягом певного часу.
Відповіді на запитання:
Ціле, оскільки число днів належить діапазону від 1 до 365.
Дійсне число, оскільки потрібно використовувати частини дня.
Символьний рядок, якщо серійний номер містить літери та цифри, або ціле число, якщо серійний номер складається лише з цифр.
Це залежить від багатьох факторів! Як вимірюється вік зразка? Кількість днів з моменту, коли його було створено (ціле число)? Дата і час (рядок)?
Виберіть дійсне число, щоб представити приблизну кількість населення за допомогою округлення (наприклад, до мільйонів), або ціле число, щоб представити точну кількість населення.
Дійсне число, оскільки результат усереднення, швидше за все, буде мати дробну частину.
Типи операцій ділення
У Python 3 оператор //
виконує ціле ділення (повертає
цілу частину результату), оператор /
виконує ділення з
плаваючою комою, та оператор ‘%’ (або модуль) повертає залишок
від цілого ділення:
ВИХІД
5 // 3: 1
5 / 3: 1.6666666666666667
5 % 3: 2
Припустимо, що num_subjects
- це кількість суб’єктів,
які беруть участь у дослідженні, а num_per_survey
—
кількість, яка може взяти участь в одному опитуванні. Напишіть вираз,
який обчислює кількість необхідних опитувань для охоплення кожного хоча
б один раз.
Потрібно визначити мінімальну кількість опитувань для охоплення
кожного суб’єкта хоча б один раз, тобто округлене значення
num_subjects/num_per_survey
. Це еквівалентно виконанню
дійсного ділення за допомогою оператору //
і додаванню 1 до
результату. Перед діленням нам потрібно відняти 1 від кількості
num_subjects
, щоб працювати з випадком, коли
num_subjects
порівну ділиться на
num_per_survey
.
PYTHON
num_subjects = 600
num_per_survey = 42
num_surveys = (num_subjects - 1) // num_per_survey + 1
print(num_subjects, 'subjects,', num_per_survey, 'per survey:', num_surveys)
ВИХІД
600 subjects, 42 per survey: 15
Перетворення рядків у числа
Якщо потрібно, функція float()
перетворить рядок у
дійсне число, а функція int()
перетворить дійсне число в
ціле:
ВИХІД
string to float: 3.4
float to int: 3
Якщо перетворення не має сенсу, то генерується повідомлення про помилку.
ПОМИЛКА
---------------------------------------------------------------------------
ValueError Traceback (most recent call last)
<ipython-input-5-df3b790bf0a2> in <module>
----> 1 print("string to float:", float("Hello world!"))
ValueError: could not convert string to float: 'Hello world!'
Беручи це до уваги, чого чекати від наступної програми?
Що вона робить насправді?
Як це пояснити?
Що ви очікуєте від цієї програми? Чому б не очікувати, що у Python 3
команда int
перетворить рядок “3.4” на 3.4 та виконає
додаткове перетворення у ціле число 3. Зрештою, Python 3 вміє робити
багато іншої “магії” - хіба це не частина його привабливості?
ВИХІД
---------------------------------------------------------------------------
ValueError Traceback (most recent call last)
<ipython-input-2-ec6729dfccdc> in <module>
----> 1 int("3.4")
ValueError: invalid literal for int() with base 10: '3.4'
Однак Python 3 видає помилку. Чому? Можливо, щоб бути послідовним. Якщо вам потрібно, щоб Python виконав два послідовних перетворення типів, ви повинні чітко вказати кожне перетворення у своєму коді.
ВИХІД
3
Арифметичні дії з різними типами
Правильні відповіді: 1 та 4
Комплексні числа
Python підтримує комплексні числа, які записуються як
1.0 + 2.0j
. Якщо val
є комплексним числом, то
до його дійсної та уявної частин можна отримати доступ за допомогою так
званої крапкової нотації через val.real
та
val.imag
.
ВИХІД
6.0
2.0
- Чому, на вашу думку, Python використовує
j
замістьi
для уявної частини? - Який результат слід очікувати від виразу
1 + 2j + 3
? - Що ви очікуєте від
4j
? А що від4 j
або4 + j
?
Стандартні математичні позначення зазвичай використовують
i
для позначення комплексного числа. Однак різні джерела свідчать про те, що це було раннє позначення, яке використовувалось в електротехніці, та зараз було б дуже складно з технічної точки зору його змінити. Stack Overflow містить додаткові пояснення та обговорення.(4+2j)
4j
абоSyntax Error: invalid syntax
. В останньому випадкуj
вважається змінною і значення виразу залежить від того, чи єj
визначеним.
Ключові моменти
- Кожне значення має тип.
- Вбудована функція
type
повертає тип значення. - Типи контролюють, які операції можна виконувати над значеннями.
- Рядки можна додавати і помножувати.
- Рядки мають довжину (але числа її не мають).
- Необхідно перетворювати числа в рядки або навпаки під час виконання певних операцій.
- Цілі та дійсні числа можна використовувати разом.
- Змінні можуть набути своє значення тільки через присвоювання.
Content from Вбудовані функції та довідка
Останнє оновлення 2025-04-03 | Редагувати цю сторінку
Огляд
Питання
- Як я можу використовувати вбудовані функції?
- Як я можу дізнатися, для чого вони призначені?
- Які помилки можуть виникнути в програмах?
Цілі
- Пояснення призначення функцій.
- Коректний виклик вбудованих функцій Python.
- Правильне використання вкладених вбудованих функцій.
- Використання довідки для відображення документації про вбудовані функції.
- Правильний опис ситуацій, в яких виникають помилки SyntaxError і NameError.
Використовуйте коментарі при створенні документації програм.
Функції можуть приймати нуль або більше аргументів.
- Ми вже знайомі з деякими функціями — тепер розглянемо їх докладніше.
- Аргумент - це значення, яке передається у функцію.
-
len
потребує тільки один аргумент. -
int
,str
таfloat
створюють нові значення з наявних. -
print
може приймати нуль або більше аргументів. -
print
без аргументів повертає порожній рядок.- Потрібно завжди використовувати дужки, навіть якщо вони порожні, щоб Python знав, що викликається функція.
ВИХІД
before
after
Кожна функція щось повертає.
- Кожен виклик функції дає певний результат.
- Якщо функція не має корисного результату для повернення, то вона
зазвичай повертає спеціальне значення
None
.None
- це об’єкт Python, який застосовується у будь-якому випадку, коли немає значення.
ВИХІД
example
result of print is None
Поширені вбудовані функції max
, min
та
round
.
- Використовуйте
max
, щоб знайти найбільше з одного або декількох значень. - Використовуйте
min
, щоб знайти найменше значення. - Обидві функції працюють як з рядками символів, так і з числами.
- Літери порівнюються у лексикографічному порядку, при цьому цифри “менші” ніж великі літери, а великі літери “менші” ніж малі.
ВИХІД
3
0
Функції працюють лише з певними аргументами (або комбінаціями аргументів).
-
max
таmin
мають приймати принаймні один аргумент.- “Найбільше значення з порожньої множини” - запит, який не має сенсу.
- Крім того, аргументи цих функцій мають бути порівнюваними.
ПОМИЛКА
TypeError Traceback (most recent call last)
<ipython-input-52-3f049acf3762> in <module>
----> 1 print(max(1, 'a'))
TypeError: '>' not supported between instances of 'str' and 'int'
Функції можуть мати значення за замовчуванням для певних аргументів.
-
round
округлює дійсне число. - За замовчуванням округлення відбувається до нуля знаків після точки.
ВИХІД
4
- Ми можемо вказати потрібну кількість десяткових знаків після крапки.
ВИХІД
3.7
Функції, приєднані до об’єктів, називаються методами
- Функції можуть набувати іншої форми, яка буде типовою для епізодів, пов’язаних з бібліотекою pandas.
- Методи мають такі дужки як функції, але з’являються в описі оператора після імені змінної.
- Деякі методи використовуються для внутрішніх операцій Python і відзначаються подвійними підкресленнями.
PYTHON
my_string = 'Hello world!' # створення об'єкта - рядка
print(len(my_string)) # функція len приймає рядок як аргумент і повертає довжину рядка
print(my_string.swapcase()) # виклик методу swapcase для об’єкта my_string
print(my_string.__len__()) # виклик внутрішнього методу __len__ для об’єкта my_string, який використовується функцією len(my_string)
ВИХІД
12
hELLO WORLD!
12
- Ви навіть можете побачити, як вони зв’язані. Вони працюють зліва направо.
PYTHON
print(my_string.isupper()) # Функція перевіряє, чи всі літери заглавні
print(my_string.upper()) # Функція перетворює всі літери на заглавні
print(my_string.upper().isupper()) # Тепер всі літери заглавні
ВИХІД
False
HELLO WORLD
True
Використовуйте вбудовану функцію help
, щоб отримати
довідку щодо функції.
- Кожна вбудована функція має онлайн-документацію.
ВИХІД
Файл допомоги щодо вбудованої функції round зі стандартної бібліотеки Python:
round(number, ndigits=None)
Round a number to a given precision in decimal digits.
The return value is an integer if ndigits is omitted or None. Otherwise
the return value has the same type as the number. ndigits may be negative.
Два шляхи отримання допомоги у Jupyter Notebook.
- Варіант 1: Помістіть курсор біля того місця, де функція викликається
в комірці (тобто біля назви функції або її параметрів),
- Утримуйте Shiftта натисніть Tab.
- Зробіть це кілька разів для розширення інформації, що повертається.
- Варіант 2: Введіть ім’я функції в комірці зі знаком питання після нього. Потім запустіть комірку.
Python повідомляє про синтаксичну помилку, коли він не може зрозуміти вихідний код програми.
- Він навіть не намагатиметься запустити програму, якщо її неможливо коректно прочитати.
ПОМИЛКА
File "<ipython-input-56-f42768451d55>", line 2
name = 'Feng
^
SyntaxError: EOL while scanning string literal
ПОМИЛКА
File "<ipython-input-57-ccc3df3cf902>", line 2
age = = 52
^
SyntaxError: invalid syntax
- Подивіться уважніше на повідомлення про помилку:
ПОМИЛКА
File "<ipython-input-6-d1cc229bf815>", line 1
print ("hello world"
^
SyntaxError: unexpected EOF while parsing
- Повідомлення вказує на проблему в першому рядку введеної програми
(“line 1”).
- У цьому випадку “ipython-input” у назві файлу повідомляє нам, що ми працюємо з IPython, тобто з інтерпретатором Python, який застосовується в Jupyter Notebook.
- Фрагмент
-6-
в назві файлу вказує на те, що помилка сталася в комірці 6. - Далі йде проблемний рядок коду, на що вказує символ
^
.
Python повідомляє про помилку виконання, коли щось йде не так під час виконання програми.
ПОМИЛКА
NameError Traceback (most recent call last)
<ipython-input-59-1214fb6c55fc> in <module>
1 age = 53
----> 2 remaining = 100 - aege # mis-spelled 'age'
NameError: name 'aege' is not defined
- Аналіз вихідного кода дозволяє виправити синтаксичні помилки, а на етапі компілювання можна виправити помилки виконання.
Порядок виконання операцій
Порядок виконання операцій:
1.1 * radiance = 1.1
1.1 - 0.5 = 0.6
min(radiance, 0.6) = 0.6
2.0 + 0.6 = 2.6
max(2.1, 2.6) = 2.6
На кінець,
radiance = 2.6
Знайдіть відмінності
- Подумайте, що виведе кожен з операторів
print
у наведеній нижче програмі. - Функція
max(len(rich), poor)
поверне відповідь або повідомлення про помилку? Якщо поверне відповідь, чи буде вона мати сенс?
ВИХІД
c
ВИХІД
tin
ВИХІД
4
max(len(rich), poor)
повертає TypeError. Помилка виникає
при виконанні max(4, 'tin')
тому що, як ми обговорювали
раніше, порівнювати рядок і ціле число не можна.
ПОМИЛКА
TypeError Traceback (most recent call last)
<ipython-input-65-bc82ad05177a> in <module>
----> 1 max(len(rich), poor)
TypeError: '>' not supported between instances of 'str' and 'int'
Чому ні?
Чому саме max
і min
не повертають
None
, коли вони викликаються без аргументів?
max
and min
повертають TypeErrors у цьому
випадку, тому що не було вказано правильну кількість параметрів. Якби
компілятор просто повернув None
, помилку було б набагато
важче відстежити, тому що це значення було б збережено в змінній і
використано пізніше в програмі.
Останній символ рядка
Якщо Python починає рахувати з нуля, та len
повертає
кількість символів у рядку, то який індекс отримає останній символ у
рядку name
? (Примітка: ми побачимо простіший спосіб зробити
це в подальшому епізоді)
name[len(name) - 1]
Вивчайте документацію Python!
Офіційна документація Python вочевидь є найповнішим джерелом інформації про мову. Вона доступна різними мовами та містить багато корисних ресурсів. Сторінка вбудованих функцій містить каталог усіх таких функцій, включаючи ті, про які ми вже говорили на цьому уроці. Деякі з них більш досконалі та на цей час зайві, але інші - дуже прості та корисні.
Ключові моменти
- Використовуйте коментарі при створенні документації програм.
- Функції можуть сприймати нуль або більше аргументів.
- Поширені вбудовані функції
max
,min
таround
. - Функції можуть працювати лише з певними аргументами (комбінаціями аргументів).
- Функції можуть мати значення за замовчуванням для певних аргументів.
- Використовуйте вбудовану функцію
help
, щоб отримати довідку щодо функції. - Є два шляхи отримання допомоги у Jupyter Notebook.
- Кожна функція щось повертає.
- Python повідомляє про синтаксичну помилку, коли він не може зрозуміти вихідний код програми.
- Python повідомляє про помилку виконання (runtime error), коли щось йде не так під час виконання програми.
- Якщо перечитаєте вихідний код, можна виправити синтаксичні помилки, а якщо відстежите дії інтерпретатора - помилки виконання.
Content from Ранкова кава
Останнє оновлення 2024-10-08 | Редагувати цю сторінку
Вправа для рефлексії
За кавою подумайте і обговоріть наступне:
- Якими є різні типи помилок в Python?
- Чи завжди код давав очікувані результати? Якщо ні, то чому?
- Чи можна щось зробити, щоб запобігти помилкам під час написання коду?
Content from Бібліотеки
Останнє оновлення 2025-04-24 | Редагувати цю сторінку
Огляд
Питання
- Як використовувати програмне забезпечення, написане іншими людьми?
- Як дізнатися, які саме функції виконує це програмне забезпечення?
Цілі
- Переваги створення та використання бібліотек програмного забезпечення.
- Імпорт та використання стандартних бібліотек Python у власних програмах.
- Пошук документації про стандартні бібліотеки в інтерактивному режимі (в інтерпретаторі) або онлайн.
Більша частина потужності мови програмування полягає в її бібліотеках.
-
Бібліотека - це колекція файлів (так званих
модулів), що містить функції для використання іншими
програмами.
- Може також визначати значення даних (наприклад, числові константи) та інші речі.
- Передбачається, що зміст бібліотеки певним чином взаємопов’язаний, але немає засобів це проконтролювати.
- Стандартна бібліотека Python — це великий набір модулів, що входить до складу базової інсталяції Python.
- Багато додаткових бібліотек доступні в PyPI (Python Package Index - репозиторій програм для Python).
- Пізніше ми побачимо, як писати нові бібліотеки.
Бібліотеки та модулі
Бібліотека — це набір модулів, але ці терміни часто вважаються взаємозамінними, особливо тому, що багато бібліотек складаються лише з одного модуля, тому не хвилюйтеся, якщо ви їх плутаєте.
Щоб використати бібліотечний модуль, його спочатку потрібно імпортувати.
- Для завантаження бібліотечного модуля в пам’ять програми
використовуйте
import
. - Потім посилайтеся на функції модуля за допомогою
module_name.function_name
.- У Python синтаксис
a.b
означає щоa
міститьb
, або іншими словами,b
є частиноюa
, абоb
належить доa
.
- У Python синтаксис
- Використовуючи
math
, один із модулів стандартної бібліотеки, маємо:
ВИХІД
pi is 3.141592653589793
cos(pi) is -1.0
- На кожен елемент модуля потрібно посилатися разом з його назвою.
-
math.cos(pi)
не спрацює: посилання наpi
жодним чином не “успадковує” посилання наmath
при виклику функціїcos()
.
-
Використовуйте help
, щоб дізнатися про вміст
бібліотечного модуля.
- Працює так само, як довідка для функції.
ВИХІД
Help on module math:
NAME
math
MODULE REFERENCE
http://docs.python.org/3/library/math
The following documentation is automatically generated from the Python
source files. It may be incomplete, incorrect or include features that
are considered implementation detail and may vary between Python
implementations. When in doubt, consult the module reference at the
location listed above.
DESCRIPTION
This module is always available. It provides access to the
mathematical functions defined by the C standard.
FUNCTIONS
acos(x, /)
Return the arc cosine (measured in radians) of x.
⋮ ⋮ ⋮
Щоб скоротити програми, імпортуйте лише певні елементи з бібліотечного модуля.
- Використовуйте
from ... import ...
, щоб завантажити лише певні елементи з бібліотечного модуля. - Потім звертайтеся до них безпосередньо без назви бібліотеки як префікса.
ВИХІД
cos(pi) is -1.0
Створіть псевдонім для бібліотечного модуля під час його імпорту для скорочення програм.
- Використовуйте
import ... ... as ...
, щоб надати бібліотеці короткий псевдонім під час її імпорту. - Потім звертайтеся до елементів у бібліотеці, використовуючи цю скорочену назву.
ВИХІД
cos(pi) is -1.0
- Зазвичай цей метод використовується для бібліотек, які дуже поширені
або мають довгі імена.
- Наприклад, бібліотека для побудови графіків
matplotlib
часто має псевдонімmpl
.
- Наприклад, бібліотека для побудови графіків
- Але цей спосіб ускладнює програми, оскільки читачі мають вивчити псевдоніми вашої програми.
Знайомство з модулем math
- За допомогою якої функції з модуля
math
можна обчислити квадратний корінь без використанняsqrt
? - Оскільки бібліотека містить цю функцію, чому існує
sqrt
?
Використовуючи
help(math)
ми бачимо, що у нас єpow(x,y)
на додаток доsqrt(x)
, отже ми можемо застосуватиpow(x, 0.5)
для визначення квадратного кореня.Функція
sqrt(x)
, ймовірно, легша для читання в вихідному коді, ніжpow(x, 0.5)
. Читабельність є основою хорошого стилю програмування, отже має сенс надати спеціальну функцію для цього конкретного поширеного випадку.
Крім того, дизайн бібліотеки math
у Python бере свій
початок у стандарті мови C, яка включає як sqrt(x)
, так і
pow(x,y)
, тож трохи історії програмування відображається в
назвах функцій Python.
Пошук правильного модуля
Припустимо, ви хочете вибрати випадковий символ з рядка:
- Який модуль зі стандартної бібліотеки може допомогти?
- Яку функцію ви б вибрали з цього модуля? Чи є альтернативи?
- Спробуйте написати програму, яка використовує цю функцію.
Здається, модуль random може допомогти.
Рядок містить 11 символів, кожен з яких має позиційний індекс від 0
до 10. Ви можете використовувати функції random.randrange
або random.randint
,
щоб отримати випадкове число від 0 до 10, а потім вибрати символ з
bases
в цій позиції:
або більш компактно:
Можливо, ви знайшли ще функцію random.sample
?
Ця функція дозволяє використовувати трохи менше коду, але може бути
трохи складнішою для розуміння при читанні:
Зверніть увагу, що ця функція повертає список (list) значень. Ми дізнаємося про списки у епізоді 11.
Найпростіше і найкоротше рішення - це функція random.choice
,
яка виконує саме те, чого ми хочемо:
Головоломка (задача Парсона). Приклад програмування
Упорядкуйте наступні оператори таким чином, щоб друкувалась випадкова основа ДНК та її індекс в рядку. Не всі оператори можуть бути потрібні. За необхідності додавайте проміжні змінні.
Коли доступна допомога?
Ваш колега виконав запит help(math)
. Python повернув
помилку:
ПОМИЛКА
NameError: name 'math' is not defined
Що забув зробити ваш колега?
Імпортувати модуль math (import math
)
можна записати як
Оскільки ви щойно написали код і знайомі з ним, вам справді легше читати першу версію. Але при спробі прочитати величезну купу коду, написаного кимось іншим, або коли повертаєтесь до свого власного величезного фрагмента коду через кілька місяців, нескорочені імена часто легші, за винятком випадку, де є чіткі умовні позначення скорочень.
Існує багато способів імпорту бібліотек!
Зіставте наступні команди друку з відповідними викликами бібліотеки.
Команди друку:
print("sin(pi/2) =", sin(pi/2))
print("sin(pi/2) =", m.sin(m.pi/2))
print("sin(pi/2) =", math.sin(math.pi/2))
Виклик бібліотеки:
from math import sin, pi
import math
import math as m
from math import *
- Виклики бібліотеки 1 та 4. Для прямого посилання на
sin
таpi
без назви бібліотеки як префікса, вам потрібно використовувати операторfrom ... import ...
. Виклик бібліотеки 1 явним чином імпортує дві функціїsin
таpi
, тоді як виклик бібліотеки 4 імпортує всі функції з модуляmath
. - Виклик бібліотеки 3. Тут
sin
таpi
посилаються на скорочену назву бібліотекиm
замістьmath
. Це стає можливим завдяки командіimport ... as ...
яка створює псевдонім дляmath
в формі короткого іменіm
. - Виклик бібліотеки 2. Тут
sin
таpi
посилаються на бібліотекуmath
за її стандартним імʼям, тому буде достатньо звичайного викликуimport ...
.
Примітка: хоча виклик бібліотеки 4 працює, імпорт
всіх імен модуля за допомогою шаблона *
не рекомендується, тому що в такому
разі невідомо, які імена з модуля використовуються в коді. Загалом,
краще робити імпорт якомога точнішим та імпортувати лише те, що
використовує ваш код. У виклику бібліотеки 1 оператор
import
явно повідомляє нам що функція sin
імпортується з модуля math
, але виклик бібліотеки 4 не
передає цю інформацію.
Скоріше за все, цю версію легше читати, оскільки вона менш насичена.
Основною причиною не використовувати цю форму імпорту є уникнення збігу
імен. Наприклад, ви б не імпортували degrees
таким чином,
якби також хотіли використовувати назву degrees
для власної
змінної або функції. Або якщо вам необхідно також імпортувати функцію з
назвою degrees
з іншої бібліотеки.
ВИХІД
---------------------------------------------------------------------------
ValueError Traceback (most recent call last)
<ipython-input-1-d72e1d780bab> in <module>
1 from math import log
----> 2 log(0)
ValueError: math domain error
Логарифм
x
визначено лише дляx > 0
, тому 0 знаходиться за межами області визначення функції.Ви отримуєте повідомлення про помилку типу
ValueError
, яке вказує на те, що функція отримала неприпустиме значення аргументу. Додаткове повідомлення “math domain error” пояснює, в чому полягає проблема.
Ключові моменти
- Більша частина потужності мови програмування полягає в її бібліотеках.
- Щоб використати бібліотечний модуль, його спочатку потрібно імпортувати.
- Використовуйте
help
, щоб дізнатися про вміст бібліотечного модуля. - Імпортуйте певні елементи з бібліотечного модуля, щоб скоротити програми.
- Створюйте псевдонім для бібліотечного модуля під час його імпорту для скорочення програм.
Content from Reading Tabular Data into DataFrames
Останнє оновлення 2025-04-24 | Редагувати цю сторінку
Огляд
Питання
- How can I read tabular data?
Цілі
- Import the Pandas library.
- Use Pandas to load a simple CSV data set.
- Get some basic information about a Pandas DataFrame.
Use the Pandas library to do statistics on tabular data.
- Pandas is a widely-used Python library for statistics, particularly on tabular data.
- Borrows many features from R’s dataframes.
- A 2-dimensional table whose columns have names and potentially have different data types.
- Load Pandas with
import pandas as pd
. The aliaspd
is commonly used to refer to the Pandas library in code. - Read a Comma Separated Values (CSV) data file with
pd.read_csv
.- Argument is the name of the file to be read.
- Returns a dataframe that you can assign to a variable
PYTHON
import pandas as pd
data_oceania = pd.read_csv('data/gapminder_gdp_oceania.csv')
print(data_oceania)
ВИХІД
country gdpPercap_1952 gdpPercap_1957 gdpPercap_1962 \
0 Australia 10039.59564 10949.64959 12217.22686
1 New Zealand 10556.57566 12247.39532 13175.67800
gdpPercap_1967 gdpPercap_1972 gdpPercap_1977 gdpPercap_1982 \
0 14526.12465 16788.62948 18334.19751 19477.00928
1 14463.91893 16046.03728 16233.71770 17632.41040
gdpPercap_1987 gdpPercap_1992 gdpPercap_1997 gdpPercap_2002 \
0 21888.88903 23424.76683 26997.93657 30687.75473
1 19007.19129 18363.32494 21050.41377 23189.80135
gdpPercap_2007
0 34435.36744
1 25185.00911
- The columns in a dataframe are the observed variables, and the rows are the observations.
- Pandas uses backslash
\
to show wrapped lines when output is too wide to fit the screen. - Using descriptive dataframe names helps us distinguish between multiple dataframes so we won’t accidentally overwrite a dataframe or read from the wrong one.
File Not Found
Our lessons store their data files in a data
sub-directory, which is why the path to the file is
data/gapminder_gdp_oceania.csv
. If you forget to include
data/
, or if you include it but your copy of the file is
somewhere else, you will get a runtime
error that ends with a line like this:
ПОМИЛКА
FileNotFoundError: [Errno 2] No such file or directory: 'data/gapminder_gdp_oceania.csv'
Use index_col
to specify that a column’s values should
be used as row headings.
- Row headings are numbers (0 and 1 in this case).
- Really want to index by country.
- Pass the name of the column to
read_csv
as itsindex_col
parameter to do this. - Naming the dataframe
data_oceania_country
tells us which region the data includes (oceania
) and how it is indexed (country
).
PYTHON
data_oceania_country = pd.read_csv('data/gapminder_gdp_oceania.csv', index_col='country')
print(data_oceania_country)
ВИХІД
gdpPercap_1952 gdpPercap_1957 gdpPercap_1962 gdpPercap_1967 \
country
Australia 10039.59564 10949.64959 12217.22686 14526.12465
New Zealand 10556.57566 12247.39532 13175.67800 14463.91893
gdpPercap_1972 gdpPercap_1977 gdpPercap_1982 gdpPercap_1987 \
country
Australia 16788.62948 18334.19751 19477.00928 21888.88903
New Zealand 16046.03728 16233.71770 17632.41040 19007.19129
gdpPercap_1992 gdpPercap_1997 gdpPercap_2002 gdpPercap_2007
country
Australia 23424.76683 26997.93657 30687.75473 34435.36744
New Zealand 18363.32494 21050.41377 23189.80135 25185.00911
Use the DataFrame.info()
method to find out more about
a dataframe.
ВИХІД
<class 'pandas.core.frame.DataFrame'>
Index: 2 entries, Australia to New Zealand
Data columns (total 12 columns):
gdpPercap_1952 2 non-null float64
gdpPercap_1957 2 non-null float64
gdpPercap_1962 2 non-null float64
gdpPercap_1967 2 non-null float64
gdpPercap_1972 2 non-null float64
gdpPercap_1977 2 non-null float64
gdpPercap_1982 2 non-null float64
gdpPercap_1987 2 non-null float64
gdpPercap_1992 2 non-null float64
gdpPercap_1997 2 non-null float64
gdpPercap_2002 2 non-null float64
gdpPercap_2007 2 non-null float64
dtypes: float64(12)
memory usage: 208.0+ bytes
- This is a
DataFrame
- Two rows named
'Australia'
and'New Zealand'
- Twelve columns, each of which has two actual 64-bit floating point
values.
- We will talk later about null values, which are used to represent missing observations.
- Uses 208 bytes of memory.
The DataFrame.columns
variable stores information about
the dataframe’s columns.
- Note that this is data, not a method. (It doesn’t have
parentheses.)
- Like
math.pi
. - So do not use
()
to try to call it.
- Like
- Called a member variable, or just member.
ВИХІД
Index(['gdpPercap_1952', 'gdpPercap_1957', 'gdpPercap_1962', 'gdpPercap_1967',
'gdpPercap_1972', 'gdpPercap_1977', 'gdpPercap_1982', 'gdpPercap_1987',
'gdpPercap_1992', 'gdpPercap_1997', 'gdpPercap_2002', 'gdpPercap_2007'],
dtype='object')
Use DataFrame.T
to transpose a dataframe.
- Sometimes want to treat columns as rows and vice versa.
- Transpose (written
.T
) doesn’t copy the data, just changes the program’s view of it. - Like
columns
, it is a member variable.
ВИХІД
country Australia New Zealand
gdpPercap_1952 10039.59564 10556.57566
gdpPercap_1957 10949.64959 12247.39532
gdpPercap_1962 12217.22686 13175.67800
gdpPercap_1967 14526.12465 14463.91893
gdpPercap_1972 16788.62948 16046.03728
gdpPercap_1977 18334.19751 16233.71770
gdpPercap_1982 19477.00928 17632.41040
gdpPercap_1987 21888.88903 19007.19129
gdpPercap_1992 23424.76683 18363.32494
gdpPercap_1997 26997.93657 21050.41377
gdpPercap_2002 30687.75473 23189.80135
gdpPercap_2007 34435.36744 25185.00911
Use DataFrame.describe()
to get summary statistics
about data.
DataFrame.describe()
gets the summary statistics of only
the columns that have numerical data. All other columns are ignored,
unless you use the argument include='all'
.
ВИХІД
gdpPercap_1952 gdpPercap_1957 gdpPercap_1962 gdpPercap_1967 \
count 2.000000 2.000000 2.000000 2.000000
mean 10298.085650 11598.522455 12696.452430 14495.021790
std 365.560078 917.644806 677.727301 43.986086
min 10039.595640 10949.649590 12217.226860 14463.918930
25% 10168.840645 11274.086022 12456.839645 14479.470360
50% 10298.085650 11598.522455 12696.452430 14495.021790
75% 10427.330655 11922.958888 12936.065215 14510.573220
max 10556.575660 12247.395320 13175.678000 14526.124650
gdpPercap_1972 gdpPercap_1977 gdpPercap_1982 gdpPercap_1987 \
count 2.00000 2.000000 2.000000 2.000000
mean 16417.33338 17283.957605 18554.709840 20448.040160
std 525.09198 1485.263517 1304.328377 2037.668013
min 16046.03728 16233.717700 17632.410400 19007.191290
25% 16231.68533 16758.837652 18093.560120 19727.615725
50% 16417.33338 17283.957605 18554.709840 20448.040160
75% 16602.98143 17809.077557 19015.859560 21168.464595
max 16788.62948 18334.197510 19477.009280 21888.889030
gdpPercap_1992 gdpPercap_1997 gdpPercap_2002 gdpPercap_2007
count 2.000000 2.000000 2.000000 2.000000
mean 20894.045885 24024.175170 26938.778040 29810.188275
std 3578.979883 4205.533703 5301.853680 6540.991104
min 18363.324940 21050.413770 23189.801350 25185.009110
25% 19628.685413 22537.294470 25064.289695 27497.598692
50% 20894.045885 24024.175170 26938.778040 29810.188275
75% 22159.406358 25511.055870 28813.266385 32122.777857
max 23424.766830 26997.936570 30687.754730 34435.367440
- Not particularly useful with just two records, but very helpful when there are thousands.
Reading Other Data
Read the data in gapminder_gdp_americas.csv
(which
should be in the same directory as
gapminder_gdp_oceania.csv
) into a variable called
data_americas
and display its summary statistics.
To read in a CSV, we use pd.read_csv
and pass the
filename 'data/gapminder_gdp_americas.csv'
to it. We also
once again pass the column name 'country'
to the parameter
index_col
in order to index by country. The summary
statistics can be displayed with the DataFrame.describe()
method.
Inspecting Data
After reading the data for the Americas, use
help(data_americas.head)
and
help(data_americas.tail)
to find out what
DataFrame.head
and DataFrame.tail
do.
- What method call will display the first three rows of this data?
- What method call will display the last three columns of this data? (Hint: you may need to change your view of the data.)
- We can check out the first five rows of
data_americas
by executingdata_americas.head()
which lets us view the beginning of the DataFrame. We can specify the number of rows we wish to see by specifying the parametern
in our call todata_americas.head()
. To view the first three rows, execute:
ВИХІД
continent gdpPercap_1952 gdpPercap_1957 gdpPercap_1962 \
country
Argentina Americas 5911.315053 6856.856212 7133.166023
Bolivia Americas 2677.326347 2127.686326 2180.972546
Brazil Americas 2108.944355 2487.365989 3336.585802
gdpPercap_1967 gdpPercap_1972 gdpPercap_1977 gdpPercap_1982 \
country
Argentina 8052.953021 9443.038526 10079.026740 8997.897412
Bolivia 2586.886053 2980.331339 3548.097832 3156.510452
Brazil 3429.864357 4985.711467 6660.118654 7030.835878
gdpPercap_1987 gdpPercap_1992 gdpPercap_1997 gdpPercap_2002 \
country
Argentina 9139.671389 9308.418710 10967.281950 8797.640716
Bolivia 2753.691490 2961.699694 3326.143191 3413.262690
Brazil 7807.095818 6950.283021 7957.980824 8131.212843
gdpPercap_2007
country
Argentina 12779.379640
Bolivia 3822.137084
Brazil 9065.800825
- To check out the last three rows of
data_americas
, we would use the command,americas.tail(n=3)
, analogous tohead()
used above. However, here we want to look at the last three columns so we need to change our view and then usetail()
. To do so, we create a new DataFrame in which rows and columns are switched:
We can then view the last three columns of americas
by
viewing the last three rows of americas_flipped
:
ВИХІД
country Argentina Bolivia Brazil Canada Chile Colombia \
gdpPercap_1997 10967.3 3326.14 7957.98 28954.9 10118.1 6117.36
gdpPercap_2002 8797.64 3413.26 8131.21 33329 10778.8 5755.26
gdpPercap_2007 12779.4 3822.14 9065.8 36319.2 13171.6 7006.58
country Costa Rica Cuba Dominican Republic Ecuador ... \
gdpPercap_1997 6677.05 5431.99 3614.1 7429.46 ...
gdpPercap_2002 7723.45 6340.65 4563.81 5773.04 ...
gdpPercap_2007 9645.06 8948.1 6025.37 6873.26 ...
country Mexico Nicaragua Panama Paraguay Peru Puerto Rico \
gdpPercap_1997 9767.3 2253.02 7113.69 4247.4 5838.35 16999.4
gdpPercap_2002 10742.4 2474.55 7356.03 3783.67 5909.02 18855.6
gdpPercap_2007 11977.6 2749.32 9809.19 4172.84 7408.91 19328.7
country Trinidad and Tobago United States Uruguay Venezuela
gdpPercap_1997 8792.57 35767.4 9230.24 10165.5
gdpPercap_2002 11460.6 39097.1 7727 8605.05
gdpPercap_2007 18008.5 42951.7 10611.5 11415.8
This shows the data that we want, but we may prefer to display three columns instead of three rows, so we can flip it back:
Note: we could have done the above in a single line of code by ‘chaining’ the commands:
Reading Files in Other Directories
The data for your current project is stored in a file called
microbes.csv
, which is located in a folder called
field_data
. You are doing analysis in a notebook called
analysis.ipynb
in a sibling folder called
thesis
:
ВИХІД
your_home_directory
+-- field_data/
| +-- microbes.csv
+-- thesis/
+-- analysis.ipynb
What value(s) should you pass to read_csv
to read
microbes.csv
in analysis.ipynb
?
We need to specify the path to the file of interest in the call to
pd.read_csv
. We first need to ‘jump’ out of the folder
thesis
using ‘../’ and then into the folder
field_data
using ‘field_data/’. Then we can specify the
filename \`microbes.csv. The result is as follows:
Writing Data
As well as the read_csv
function for reading data from a
file, Pandas provides a to_csv
function to write dataframes
to files. Applying what you’ve learned about reading from files, write
one of your dataframes to a file called processed.csv
. You
can use help
to get information on how to use
to_csv
.
In order to write the DataFrame data_americas
to a file
called processed.csv
, execute the following command:
For help on read_csv
or to_csv
, you could
execute, for example:
Note that help(to_csv)
or help(pd.to_csv)
throws an error! This is due to the fact that to_csv
is not
a global Pandas function, but a member function of DataFrames. This
means you can only call it on an instance of a DataFrame e.g.,
data_americas.to_csv
or
data_oceania.to_csv
Ключові моменти
- Use the Pandas library to get basic statistics out of tabular data.
- Use
index_col
to specify that a column’s values should be used as row headings. - Use
DataFrame.info
to find out more about a dataframe. - The
DataFrame.columns
variable stores information about the dataframe’s columns. - Use
DataFrame.T
to transpose a dataframe. - Use
DataFrame.describe
to get summary statistics about data.
Content from Pandas DataFrames
Останнє оновлення 2025-04-03 | Редагувати цю сторінку
Огляд
Питання
- How can I do statistical analysis of tabular data?
Цілі
- Select individual values from a Pandas dataframe.
- Select entire rows or entire columns from a dataframe.
- Select a subset of both rows and columns from a dataframe in a single operation.
- Select a subset of a dataframe by a single Boolean criterion.
Note about Pandas DataFrames/Series
A DataFrame is a collection of Series; The DataFrame is the way Pandas represents a table, and Series is the data-structure Pandas use to represent a column.
Pandas is built on top of the Numpy library, which in practice means that most of the methods defined for Numpy Arrays apply to Pandas Series/DataFrames.
What makes Pandas so attractive is the powerful interface to access individual records of the table, proper handling of missing values, and relational-databases operations between DataFrames.
Selecting values
To access a value at the position [i,j]
of a DataFrame,
we have two options, depending on what is the meaning of i
in use. Remember that a DataFrame provides an index as a way to
identify the rows of the table; a row, then, has a position
inside the table as well as a label, which uniquely identifies
its entry in the DataFrame.
Use DataFrame.iloc[..., ...]
to select values by their
(entry) position
- Can specify location by numerical index analogously to 2D version of character selection in strings.
PYTHON
import pandas as pd
data = pd.read_csv('data/gapminder_gdp_europe.csv', index_col='country')
print(data.iloc[0, 0])
ВИХІД
1601.056136
Use DataFrame.loc[..., ...]
to select values by their
(entry) label.
- Can specify location by row and/or column name.
ВИХІД
1601.056136
Use :
on its own to mean all columns or all rows.
- Just like Python’s usual slicing notation.
ВИХІД
gdpPercap_1952 1601.056136
gdpPercap_1957 1942.284244
gdpPercap_1962 2312.888958
gdpPercap_1967 2760.196931
gdpPercap_1972 3313.422188
gdpPercap_1977 3533.003910
gdpPercap_1982 3630.880722
gdpPercap_1987 3738.932735
gdpPercap_1992 2497.437901
gdpPercap_1997 3193.054604
gdpPercap_2002 4604.211737
gdpPercap_2007 5937.029526
Name: Albania, dtype: float64
- Would get the same result printing
data.loc["Albania"]
(without a second index).
ВИХІД
country
Albania 1601.056136
Austria 6137.076492
Belgium 8343.105127
⋮ ⋮ ⋮
Switzerland 14734.232750
Turkey 1969.100980
United Kingdom 9979.508487
Name: gdpPercap_1952, dtype: float64
- Would get the same result printing
data["gdpPercap_1952"]
- Also get the same result printing
data.gdpPercap_1952
(not recommended, because easily confused with.
notation for methods)
Select multiple columns or rows using DataFrame.loc
and
a named slice.
ВИХІД
gdpPercap_1962 gdpPercap_1967 gdpPercap_1972
country
Italy 8243.582340 10022.401310 12269.273780
Montenegro 4649.593785 5907.850937 7778.414017
Netherlands 12790.849560 15363.251360 18794.745670
Norway 13450.401510 16361.876470 18965.055510
Poland 5338.752143 6557.152776 8006.506993
In the above code, we discover that slicing using
loc
is inclusive at both ends, which differs from
slicing using iloc
, where slicing
indicates everything up to but not including the final index.
Result of slicing can be used in further operations.
- Usually don’t just print a slice.
- All the statistical operators that work on entire dataframes work the same way on slices.
- E.g., calculate max of a slice.
ВИХІД
gdpPercap_1962 13450.40151
gdpPercap_1967 16361.87647
gdpPercap_1972 18965.05551
dtype: float64
ВИХІД
gdpPercap_1962 4649.593785
gdpPercap_1967 5907.850937
gdpPercap_1972 7778.414017
dtype: float64
Use comparisons to select data based on value.
- Comparison is applied element by element.
- Returns a similarly-shaped dataframe of
True
andFalse
.
PYTHON
# Use a subset of data to keep output readable.
subset = data.loc['Italy':'Poland', 'gdpPercap_1962':'gdpPercap_1972']
print('Subset of data:\n', subset)
# Which values were greater than 10000 ?
print('\nWhere are values large?\n', subset > 10000)
ВИХІД
Subset of data:
gdpPercap_1962 gdpPercap_1967 gdpPercap_1972
country
Italy 8243.582340 10022.401310 12269.273780
Montenegro 4649.593785 5907.850937 7778.414017
Netherlands 12790.849560 15363.251360 18794.745670
Norway 13450.401510 16361.876470 18965.055510
Poland 5338.752143 6557.152776 8006.506993
Where are values large?
gdpPercap_1962 gdpPercap_1967 gdpPercap_1972
country
Italy False True True
Montenegro False False False
Netherlands True True True
Norway True True True
Poland False False False
Select values or NaN using a Boolean mask.
- A frame full of Booleans is sometimes called a mask because of how it can be used.
ВИХІД
gdpPercap_1962 gdpPercap_1967 gdpPercap_1972
country
Italy NaN 10022.40131 12269.27378
Montenegro NaN NaN NaN
Netherlands 12790.84956 15363.25136 18794.74567
Norway 13450.40151 16361.87647 18965.05551
Poland NaN NaN NaN
- Get the value where the mask is true, and NaN (Not a Number) where it is false.
- Useful because NaNs are ignored by operations like max, min, average, etc.
ВИХІД
gdpPercap_1962 gdpPercap_1967 gdpPercap_1972
count 2.000000 3.000000 3.000000
mean 13120.625535 13915.843047 16676.358320
std 466.373656 3408.589070 3817.597015
min 12790.849560 10022.401310 12269.273780
25% 12955.737547 12692.826335 15532.009725
50% 13120.625535 15363.251360 18794.745670
75% 13285.513523 15862.563915 18879.900590
max 13450.401510 16361.876470 18965.055510
Group By: split-apply-combine
::::::::::::::::::::::::::::::::::::: instructor Learners often struggle here, many may not work with financial data and concepts so they find the example concepts difficult to get their head around. The biggest problem though is the line generating the wealth_score, this step needs to be talked through throughly:
- It uses implicit conversion between boolean and float values which has not been covered in the course so far.
- The axis=1 argument needs to be explained clearly. :::::::::::::::::::::::::::::::::::::::::::::::::
Pandas vectorizing methods and grouping operations are features that provide users much flexibility to analyse their data.
For instance, let’s say we want to have a clearer view on how the European countries split themselves according to their GDP.
- We may have a glance by splitting the countries in two groups during the years surveyed, those who presented a GDP higher than the European average and those with a lower GDP.
- We then estimate a wealthy score based on the historical (from 1962 to 2007) values, where we account how many times a country has participated in the groups of lower or higher GDP
PYTHON
mask_higher = data > data.mean()
wealth_score = mask_higher.aggregate('sum', axis=1) / len(data.columns)
print(wealth_score)
ВИХІД
country
Albania 0.000000
Austria 1.000000
Belgium 1.000000
Bosnia and Herzegovina 0.000000
Bulgaria 0.000000
Croatia 0.000000
Czech Republic 0.500000
Denmark 1.000000
Finland 1.000000
France 1.000000
Germany 1.000000
Greece 0.333333
Hungary 0.000000
Iceland 1.000000
Ireland 0.333333
Italy 0.500000
Montenegro 0.000000
Netherlands 1.000000
Norway 1.000000
Poland 0.000000
Portugal 0.000000
Romania 0.000000
Serbia 0.000000
Slovak Republic 0.000000
Slovenia 0.333333
Spain 0.333333
Sweden 1.000000
Switzerland 1.000000
Turkey 0.000000
United Kingdom 1.000000
dtype: float64
Finally, for each group in the wealth_score
table, we
sum their (financial) contribution across the years surveyed using
chained methods:
ВИХІД
gdpPercap_1952 gdpPercap_1957 gdpPercap_1962 gdpPercap_1967 \
0.000000 36916.854200 46110.918793 56850.065437 71324.848786
0.333333 16790.046878 20942.456800 25744.935321 33567.667670
0.500000 11807.544405 14505.000150 18380.449470 21421.846200
1.000000 104317.277560 127332.008735 149989.154201 178000.350040
gdpPercap_1972 gdpPercap_1977 gdpPercap_1982 gdpPercap_1987 \
0.000000 88569.346898 104459.358438 113553.768507 119649.599409
0.333333 45277.839976 53860.456750 59679.634020 64436.912960
0.500000 25377.727380 29056.145370 31914.712050 35517.678220
1.000000 215162.343140 241143.412730 263388.781960 296825.131210
gdpPercap_1992 gdpPercap_1997 gdpPercap_2002 gdpPercap_2007
0.000000 92380.047256 103772.937598 118590.929863 149577.357928
0.333333 67918.093220 80876.051580 102086.795210 122803.729520
0.500000 36310.666080 40723.538700 45564.308390 51403.028210
1.000000 315238.235970 346930.926170 385109.939210 427850.333420
Selection of Individual Values
No, they do not produce the same output! The output of the first statement is:
ВИХІД
gdpPercap_1952 gdpPercap_1957
country
Albania 1601.056136 1942.284244
Austria 6137.076492 8842.598030
The second statement gives:
ВИХІД
gdpPercap_1952 gdpPercap_1957 gdpPercap_1962
country
Albania 1601.056136 1942.284244 2312.888958
Austria 6137.076492 8842.598030 10750.721110
Belgium 8343.105127 9714.960623 10991.206760
Clearly, the second statement produces an additional column and an
additional row compared to the first statement.
What conclusion can we draw? We see that a numerical slice, 0:2,
omits the final index (i.e. index 2) in the range provided,
while a named slice, ‘gdpPercap_1952’:‘gdpPercap_1962’,
includes the final element.
Reconstructing Data
Explain what each line in the following short program does: what is
in first
, second
, etc.?
Let’s go through this piece of code line by line.
This line loads the dataset containing the GDP data from all
countries into a dataframe called first
. The
index_col='country'
parameter selects which column to use
as the row labels in the dataframe.
This line makes a selection: only those rows of first
for which the ‘continent’ column matches ‘Americas’ are extracted.
Notice how the Boolean expression inside the brackets,
first['continent'] == 'Americas'
, is used to select only
those rows where the expression is true. Try printing this expression!
Can you print also its individual True/False elements? (hint: first
assign the expression to a variable)
As the syntax suggests, this line drops the row from
second
where the label is ‘Puerto Rico’. The resulting
dataframe third
has one row less than the original
dataframe second
.
Again we apply the drop function, but in this case we are dropping
not a row but a whole column. To accomplish this, we need to specify
also the axis
parameter (we want to drop the second column
which has index 1).
The final step is to write the data that we have been working on to a
csv file. Pandas makes this easy with the to_csv()
function. The only required argument to the function is the filename.
Note that the file will be written in the directory from which you
started the Jupyter or Python session.
For each column in data
, idxmin
will return
the index value corresponding to each column’s minimum;
idxmax
will do accordingly the same for each column’s
maximum value.
You can use these functions whenever you want to get the row index of the minimum/maximum value and not the actual minimum/maximum value.
Practice with Selection
Assume Pandas has been imported and the Gapminder GDP data for Europe has been loaded. Write an expression to select each of the following:
- GDP per capita for all countries in 1982.
- GDP per capita for Denmark for all years.
- GDP per capita for all countries for years after 1985.
- GDP per capita for each country in 2007 as a multiple of GDP per capita for that country in 1952.
1:
2:
3:
Pandas is smart enough to recognize the number at the end of the
column label and does not give you an error, although no column named
gdpPercap_1985
actually exists. This is useful if new
columns are added to the CSV file later.
4:
Many Ways of Access
There are at least two ways of accessing a value or slice of a
DataFrame: by name or index. However, there are many others. For
example, a single column or row can be accessed either as a
DataFrame
or a Series
object.
Suggest different ways of doing the following operations on a DataFrame:
- Access a single column
- Access a single row
- Access an individual DataFrame element
- Access several columns
- Access several rows
- Access a subset of specific rows and columns
- Access a subset of row and column ranges
1. Access a single column:
PYTHON
# by name
data["col_name"] # as a Series
data[["col_name"]] # as a DataFrame
# by name using .loc
data.T.loc["col_name"] # as a Series
data.T.loc[["col_name"]].T # as a DataFrame
# Dot notation (Series)
data.col_name
# by index (iloc)
data.iloc[:, col_index] # as a Series
data.iloc[:, [col_index]] # as a DataFrame
# using a mask
data.T[data.T.index == "col_name"].T
2. Access a single row:
PYTHON
# by name using .loc
data.loc["row_name"] # as a Series
data.loc[["row_name"]] # as a DataFrame
# by name
data.T["row_name"] # as a Series
data.T[["row_name"]].T # as a DataFrame
# by index
data.iloc[row_index] # as a Series
data.iloc[[row_index]] # as a DataFrame
# using mask
data[data.index == "row_name"]
3. Access an individual DataFrame element:
PYTHON
# by column/row names
data["column_name"]["row_name"] # as a Series
data[["col_name"]].loc["row_name"] # as a Series
data[["col_name"]].loc[["row_name"]] # as a DataFrame
data.loc["row_name"]["col_name"] # as a value
data.loc[["row_name"]]["col_name"] # as a Series
data.loc[["row_name"]][["col_name"]] # as a DataFrame
data.loc["row_name", "col_name"] # as a value
data.loc[["row_name"], "col_name"] # as a Series. Preserves index. Column name is moved to `.name`.
data.loc["row_name", ["col_name"]] # as a Series. Index is moved to `.name.` Sets index to column name.
data.loc[["row_name"], ["col_name"]] # as a DataFrame (preserves original index and column name)
# by column/row names: Dot notation
data.col_name.row_name
# by column/row indices
data.iloc[row_index, col_index] # as a value
data.iloc[[row_index], col_index] # as a Series. Preserves index. Column name is moved to `.name`
data.iloc[row_index, [col_index]] # as a Series. Index is moved to `.name.` Sets index to column name.
data.iloc[[row_index], [col_index]] # as a DataFrame (preserves original index and column name)
# column name + row index
data["col_name"][row_index]
data.col_name[row_index]
data["col_name"].iloc[row_index]
# column index + row name
data.iloc[:, [col_index]].loc["row_name"] # as a Series
data.iloc[:, [col_index]].loc[["row_name"]] # as a DataFrame
# using masks
data[data.index == "row_name"].T[data.T.index == "col_name"].T
4. Access several columns:
PYTHON
# by name
data[["col1", "col2", "col3"]]
data.loc[:, ["col1", "col2", "col3"]]
# by index
data.iloc[:, [col1_index, col2_index, col3_index]]
5. Access several rows
PYTHON
# by name
data.loc[["row1", "row2", "row3"]]
# by index
data.iloc[[row1_index, row2_index, row3_index]]
6. Access a subset of specific rows and columns
PYTHON
# by names
data.loc[["row1", "row2", "row3"], ["col1", "col2", "col3"]]
# by indices
data.iloc[[row1_index, row2_index, row3_index], [col1_index, col2_index, col3_index]]
# column names + row indices
data[["col1", "col2", "col3"]].iloc[[row1_index, row2_index, row3_index]]
# column indices + row names
data.iloc[:, [col1_index, col2_index, col3_index]].loc[["row1", "row2", "row3"]]
7. Access a subset of row and column ranges
PYTHON
# by name
data.loc["row1":"row2", "col1":"col2"]
# by index
data.iloc[row1_index:row2_index, col1_index:col2_index]
# column names + row indices
data.loc[:, "col1_name":"col2_name"].iloc[row1_index:row2_index]
# column indices + row names
data.iloc[:, col1_index:col2_index].loc["row1":"row2"]
Exploring available methods using the
dir()
function
Python includes a dir()
function that can be used to
display all of the available methods (functions) that are built into a
data object. In Episode 4, we used some methods with a string. But we
can see many more are available by using dir()
:
This command returns:
PYTHON
['__add__',
...
'__subclasshook__',
'capitalize',
'casefold',
'center',
...
'upper',
'zfill']
You can use help()
or Shift+Tab to
get more information about what these methods do.
Assume Pandas has been imported and the Gapminder GDP data for Europe
has been loaded as data
. Then, use dir()
to
find the function that prints out the median per-capita GDP across all
European countries for each year that information is available.
Interpretation
Poland’s borders have been stable since 1945, but changed several times in the years before then. How would you handle this if you were creating a table of GDP per capita for Poland for the entire twentieth century?
Ключові моменти
- Use
DataFrame.iloc[..., ...]
to select values by integer location. - Use
:
on its own to mean all columns or all rows. - Select multiple columns or rows using
DataFrame.loc
and a named slice. - Result of slicing can be used in further operations.
- Use comparisons to select data based on value.
- Select values or NaN using a Boolean mask.
Content from Plotting
Останнє оновлення 2025-01-24 | Редагувати цю сторінку
Огляд
Питання
- How can I plot my data?
- How can I save my plot for publishing?
Цілі
- Create a time series plot showing a single data set.
- Create a scatter plot showing relationship between two data sets.
matplotlib
is the
most widely used scientific plotting library in Python.
- Commonly use a sub-library called
matplotlib.pyplot
. - The Jupyter Notebook will render plots inline by default.
- Simple plots are then (fairly) simple to create.
PYTHON
time = [0, 1, 2, 3]
position = [0, 100, 200, 300]
plt.plot(time, position)
plt.xlabel('Time (hr)')
plt.ylabel('Position (km)')
Display All Open Figures
In our Jupyter Notebook example, running the cell should generate the figure directly below the code. The figure is also included in the Notebook document for future viewing. However, other Python environments like an interactive Python session started from a terminal or a Python script executed via the command line require an additional command to display the figure.
Instruct matplotlib
to show a figure:
This command can also be used within a Notebook - for instance, to display multiple figures if several are created by a single cell.
Plot data directly from a Pandas dataframe
.
- We can also plot Pandas dataframes.
- Before plotting, we convert the column headings from a
string
tointeger
data type, since they represent numerical values, using str.replace() to remove thegpdPercap_
prefix and then astype(int) to convert the series of string values (['1952', '1957', ..., '2007']
) to a series of integers:[1925, 1957, ..., 2007]
.
PYTHON
import pandas as pd
data = pd.read_csv('data/gapminder_gdp_oceania.csv', index_col='country')
# Extract year from last 4 characters of each column name
# The current column names are structured as 'gdpPercap_(year)',
# so we want to keep the (year) part only for clarity when plotting GDP vs. years
# To do this we use replace(), which removes from the string the characters stated in the argument
# This method works on strings, so we use replace() from Pandas Series.str vectorized string functions
years = data.columns.str.replace('gdpPercap_', '')
# Convert year values to integers, saving results back to dataframe
data.columns = years.astype(int)
data.loc['Australia'].plot()
Select and transform data, then plot it.
- By default,
DataFrame.plot
plots with the rows as the X axis. - We can transpose the data in order to plot multiple series.
Many styles of plot are available.
- For example, do a bar plot using a fancier style.
Data can also be plotted by calling the matplotlib
plot
function directly.
- The command is
plt.plot(x, y)
- The color and format of markers can also be specified as an
additional optional argument e.g.,
b-
is a blue line,g--
is a green dashed line.
Get Australia data from dataframe
PYTHON
years = data.columns
gdp_australia = data.loc['Australia']
plt.plot(years, gdp_australia, 'g--')
Can plot many sets of data together.
PYTHON
# Select two countries' worth of data.
gdp_australia = data.loc['Australia']
gdp_nz = data.loc['New Zealand']
# Plot with differently-colored markers.
plt.plot(years, gdp_australia, 'b-', label='Australia')
plt.plot(years, gdp_nz, 'g-', label='New Zealand')
# Create legend.
plt.legend(loc='upper left')
plt.xlabel('Year')
plt.ylabel('GDP per capita ($)')
Adding a Legend
Often when plotting multiple datasets on the same figure it is desirable to have a legend describing the data.
This can be done in matplotlib
in two stages:
- Provide a label for each dataset in the figure:
PYTHON
plt.plot(years, gdp_australia, label='Australia')
plt.plot(years, gdp_nz, label='New Zealand')
- Instruct
matplotlib
to create the legend.
By default matplotlib will attempt to place the legend in a suitable
position. If you would rather specify a position this can be done with
the loc=
argument, e.g to place the legend in the upper
left corner of the plot, specify loc='upper left'
- Plot a scatter plot correlating the GDP of Australia and New Zealand
- Use either
plt.scatter
orDataFrame.plot.scatter
Minima and Maxima
Fill in the blanks below to plot the minimum GDP per capita over time for all the countries in Europe. Modify it again to plot the maximum GDP per capita over time for Europe.
Correlations
Modify the example in the notes to create a scatter plot showing the relationship between the minimum and maximum GDP per capita among the countries in Asia for each year in the data set. What relationship do you see (if any)?
PYTHON
data_asia = pd.read_csv('data/gapminder_gdp_asia.csv', index_col='country')
data_asia.describe().T.plot(kind='scatter', x='min', y='max')
No particular correlations can be seen between the minimum and maximum GDP values year on year. It seems the fortunes of asian countries do not rise and fall together.
Correlations (continued)
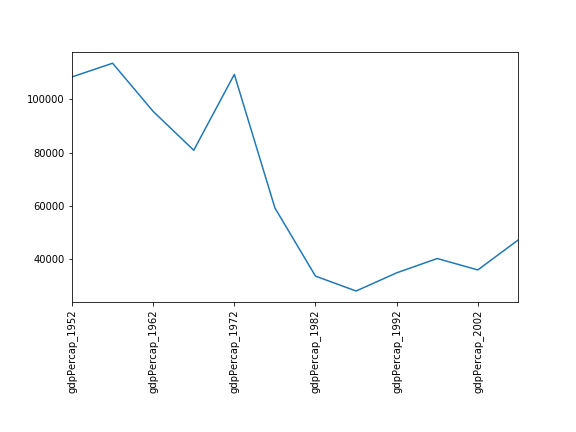
Seems the variability in this value is due to a sharp drop after 1972. Some geopolitics at play perhaps? Given the dominance of oil producing countries, maybe the Brent crude index would make an interesting comparison? Whilst Myanmar consistently has the lowest GDP, the highest GDP nation has varied more notably.
More Correlations
This short program creates a plot showing the correlation between GDP and life expectancy for 2007, normalizing marker size by population:
PYTHON
data_all = pd.read_csv('data/gapminder_all.csv', index_col='country')
data_all.plot(kind='scatter', x='gdpPercap_2007', y='lifeExp_2007',
s=data_all['pop_2007']/1e6)
Using online help and other resources, explain what each argument to
plot
does.
A good place to look is the documentation for the plot function - help(data_all.plot).
kind - As seen already this determines the kind of plot to be drawn.
x and y - A column name or index that determines what data will be placed on the x and y axes of the plot
s - Details for this can be found in the documentation of plt.scatter. A single number or one value for each data point. Determines the size of the plotted points.
Saving your plot to a file
If you are satisfied with the plot you see you may want to save it to a file, perhaps to include it in a publication. There is a function in the matplotlib.pyplot module that accomplishes this: savefig. Calling this function, e.g. with
will save the current figure to the file my_figure.png
.
The file format will automatically be deduced from the file name
extension (other formats are pdf, ps, eps and svg).
Note that functions in plt
refer to a global figure
variable and after a figure has been displayed to the screen (e.g. with
plt.show
) matplotlib will make this variable refer to a new
empty figure. Therefore, make sure you call plt.savefig
before the plot is displayed to the screen, otherwise you may find a
file with an empty plot.
When using dataframes, data is often generated and plotted to screen
in one line. In addition to using plt.savefig
, we can save
a reference to the current figure in a local variable (with
plt.gcf
) and call the savefig
class method
from that variable to save the figure to file.
Making your plots accessible
Whenever you are generating plots to go into a paper or a presentation, there are a few things you can do to make sure that everyone can understand your plots.
- Always make sure your text is large enough to read. Use the
fontsize
parameter inxlabel
,ylabel
,title
, andlegend
, andtick_params
withlabelsize
to increase the text size of the numbers on your axes. - Similarly, you should make your graph elements easy to see. Use
s
to increase the size of your scatterplot markers andlinewidth
to increase the sizes of your plot lines. - Using color (and nothing else) to distinguish between different plot
elements will make your plots unreadable to anyone who is colorblind, or
who happens to have a black-and-white office printer. For lines, the
linestyle
parameter lets you use different types of lines. For scatterplots,marker
lets you change the shape of your points. If you’re unsure about your colors, you can use Coblis or Color Oracle to simulate what your plots would look like to those with colorblindness.
Ключові моменти
-
matplotlib
is the most widely used scientific plotting library in Python. - Plot data directly from a Pandas dataframe.
- Select and transform data, then plot it.
- Many styles of plot are available: see the Python Graph Gallery for more options.
- Can plot many sets of data together.
Content from Ланч
Останнє оновлення 2025-02-23 | Редагувати цю сторінку
За обідом поміркуйте та обговоріть наступне:
- Які пакети можна використовувати в Python, і чому ми їх використовуємо?
- How would data need to be formatted to be used in Pandas data frames? Would the data you have meet these requirements?
- What limitations or problems might you run into when thinking about how to apply what we’ve learned to your own projects or data?
Content from Lists
Останнє оновлення 2024-07-02 | Редагувати цю сторінку
Огляд
Питання
- How can I store multiple values?
Цілі
- Explain why programs need collections of values.
- Write programs that create flat lists, index them, slice them, and modify them through assignment and method calls.
A list stores many values in a single structure.
- Doing calculations with a hundred variables called
pressure_001
,pressure_002
, etc., would be at least as slow as doing them by hand. - Use a list to store many values together.
- Contained within square brackets
[...]
. - Values separated by commas
,
.
- Contained within square brackets
- Use
len
to find out how many values are in a list.
PYTHON
pressures = [0.273, 0.275, 0.277, 0.275, 0.276]
print('pressures:', pressures)
print('length:', len(pressures))
ВИХІД
pressures: [0.273, 0.275, 0.277, 0.275, 0.276]
length: 5
Use an item’s index to fetch it from a list.
- Just like strings.
PYTHON
print('zeroth item of pressures:', pressures[0])
print('fourth item of pressures:', pressures[4])
ВИХІД
zeroth item of pressures: 0.273
fourth item of pressures: 0.276
Lists’ values can be replaced by assigning to them.
- Use an index expression on the left of assignment to replace a value.
ВИХІД
pressures is now: [0.265, 0.275, 0.277, 0.275, 0.276]
Appending items to a list lengthens it.
- Use
list_name.append
to add items to the end of a list.
PYTHON
primes = [2, 3, 5]
print('primes is initially:', primes)
primes.append(7)
print('primes has become:', primes)
ВИХІД
primes is initially: [2, 3, 5]
primes has become: [2, 3, 5, 7]
-
append
is a method of lists.- Like a function, but tied to a particular object.
- Use
object_name.method_name
to call methods.- Deliberately resembles the way we refer to things in a library.
- We will meet other methods of lists as we go along.
- Use
help(list)
for a preview.
- Use
-
extend
is similar toappend
, but it allows you to combine two lists. For example:
PYTHON
teen_primes = [11, 13, 17, 19]
middle_aged_primes = [37, 41, 43, 47]
print('primes is currently:', primes)
primes.extend(teen_primes)
print('primes has now become:', primes)
primes.append(middle_aged_primes)
print('primes has finally become:', primes)
ВИХІД
primes is currently: [2, 3, 5, 7]
primes has now become: [2, 3, 5, 7, 11, 13, 17, 19]
primes has finally become: [2, 3, 5, 7, 11, 13, 17, 19, [37, 41, 43, 47]]
Note that while extend
maintains the “flat” structure of
the list, appending a list to a list means the last element in
primes
will itself be a list, not an integer. Lists can
contain values of any type; therefore, lists of lists are possible.
Use del
to remove items from a list entirely.
- We use
del list_name[index]
to remove an element from a list (in the example, 9 is not a prime number) and thus shorten it. -
del
is not a function or a method, but a statement in the language.
PYTHON
primes = [2, 3, 5, 7, 9]
print('primes before removing last item:', primes)
del primes[4]
print('primes after removing last item:', primes)
ВИХІД
primes before removing last item: [2, 3, 5, 7, 9]
primes after removing last item: [2, 3, 5, 7]
The empty list contains no values.
- Use
[]
on its own to represent a list that doesn’t contain any values.- “The zero of lists.”
- Helpful as a starting point for collecting values (which we will see in the next episode).
Lists may contain values of different types.
- A single list may contain numbers, strings, and anything else.
Character strings can be indexed like lists.
- Get single characters from a character string using indexes in square brackets.
PYTHON
element = 'carbon'
print('zeroth character:', element[0])
print('third character:', element[3])
ВИХІД
zeroth character: c
third character: b
Character strings are immutable.
- Cannot change the characters in a string after it has been created.
- Immutable: can’t be changed after creation.
- In contrast, lists are mutable: they can be modified in place.
- Python considers the string to be a single value with parts, not a collection of values.
ПОМИЛКА
TypeError: 'str' object does not support item assignment
- Lists and character strings are both collections.
Indexing beyond the end of the collection is an error.
- Python reports an
IndexError
if we attempt to access a value that doesn’t exist.- This is a kind of runtime error.
- Cannot be detected as the code is parsed because the index might be calculated based on data.
ВИХІД
IndexError: string index out of range
How Large is a Slice?
If start
and stop
are both non-negative
integers, how long is the list values[start:stop]
?
The list values[start:stop]
has up to
stop - start
elements. For example,
values[1:4]
has the 3 elements values[1]
,
values[2]
, and values[3]
. Why ‘up to’? As we
saw in episode 2, if stop
is greater than the total length of the list values
, we
will still get a list back but it will be shorter than expected.
list('some string')
converts a string into a list containing all of its characters.join
returns a string that is the concatenation of each string element in the list and adds the separator between each element in the list. This results inx-y-z
. The separator between the elements is the string that provides this method.
Working With the End
What does the following program print?
- How does Python interpret a negative index?
- If a list or string has N elements, what is the most negative index that can safely be used with it, and what location does that index represent?
- If
values
is a list, what doesdel values[-1]
do? - How can you display all elements but the last one without changing
values
? (Hint: you will need to combine slicing and negative indexing.)
The program prints m
.
Python interprets a negative index as starting from the end (as opposed to starting from the beginning). The last element is
-1
.The last index that can safely be used with a list of N elements is element
-N
, which represents the first element.del values[-1]
removes the last element from the list.values[:-1]
The program prints
stride
is the step size of the slice.The slice
1::2
selects all even-numbered items from a collection: it starts with element1
(which is the second element, since indexing starts at0
), goes on until the end (since noend
is given), and uses a step size of2
(i.e., selects every second element).
ВИХІД
lithium
The first statement prints the whole string, since the slice goes beyond the total length of the string. The second statement returns an empty string, because the slice goes “out of bounds” of the string.
Program A prints
ВИХІД
letters is ['g', 'o', 'l', 'd'] and result is ['d', 'g', 'l', 'o']
Program B prints
ВИХІД
letters is ['d', 'g', 'l', 'o'] and result is None
sorted(letters)
returns a sorted copy of the list
letters
(the original list letters
remains
unchanged), while letters.sort()
sorts the list
letters
in-place and does not return anything.
Program A prints
ВИХІД
new is ['D', 'o', 'l', 'd'] and old is ['D', 'o', 'l', 'd']
Program B prints
ВИХІД
new is ['D', 'o', 'l', 'd'] and old is ['g', 'o', 'l', 'd']
new = old
makes new
a reference to the list
old
; new
and old
point towards
the same object.
new = old[:]
however creates a new list object
new
containing all elements from the list old
;
new
and old
are different objects.
Ключові моменти
- A list stores many values in a single structure.
- Use an item’s index to fetch it from a list.
- Lists’ values can be replaced by assigning to them.
- Appending items to a list lengthens it.
- Use
del
to remove items from a list entirely. - The empty list contains no values.
- Lists may contain values of different types.
- Character strings can be indexed like lists.
- Character strings are immutable.
- Indexing beyond the end of the collection is an error.
Content from For Loops
Останнє оновлення 2024-07-02 | Редагувати цю сторінку
Огляд
Питання
- How can I make a program do many things?
Цілі
- Explain what for loops are normally used for.
- Trace the execution of a simple (unnested) loop and correctly state the values of variables in each iteration.
- Write for loops that use the Accumulator pattern to aggregate values.
A for loop executes commands once for each value in a collection.
- Doing calculations on the values in a list one by one is as painful
as working with
pressure_001
,pressure_002
, etc. - A for loop tells Python to execute some statements once for each value in a list, a character string, or some other collection.
- “for each thing in this group, do these operations”
- This
for
loop is equivalent to:
- And the
for
loop’s output is:
ВИХІД
2
3
5
A for
loop is made up of a collection, a loop variable,
and a body.
- The collection,
[2, 3, 5]
, is what the loop is being run on. - The body,
print(number)
, specifies what to do for each value in the collection. - The loop variable,
number
, is what changes for each iteration of the loop.- The “current thing”.
The first line of the for
loop must end with a colon,
and the body must be indented.
- The colon at the end of the first line signals the start of a block of statements.
- Python uses indentation rather than
{}
orbegin
/end
to show nesting.- Any consistent indentation is legal, but almost everyone uses four spaces.
ПОМИЛКА
IndentationError: expected an indented block
- Indentation is always meaningful in Python.
ПОМИЛКА
File "<ipython-input-7-f65f2962bf9c>", line 2
lastName = "Smith"
^
IndentationError: unexpected indent
- This error can be fixed by removing the extra spaces at the beginning of the second line.
Loop variables can be called anything.
- As with all variables, loop variables are:
- Created on demand.
- Meaningless: their names can be anything at all.
The body of a loop can contain many statements.
- But no loop should be more than a few lines long.
- Hard for human beings to keep larger chunks of code in mind.
ВИХІД
2 4 8
3 9 27
5 25 125
Use range
to iterate over a sequence of numbers.
- The built-in function
range
produces a sequence of numbers.- Not a list: the numbers are produced on demand to make looping over large ranges more efficient.
-
range(N)
is the numbers 0..N-1- Exactly the legal indices of a list or character string of length N
ВИХІД
a range is not a list: range(0, 3)
0
1
2
The Accumulator pattern turns many values into one.
- A common pattern in programs is to:
- Initialize an accumulator variable to zero, the empty string, or the empty list.
- Update the variable with values from a collection.
PYTHON
# Sum the first 10 integers.
total = 0
for number in range(10):
total = total + (number + 1)
print(total)
ВИХІД
55
- Read
total = total + (number + 1)
as:- Add 1 to the current value of the loop variable
number
. - Add that to the current value of the accumulator variable
total
. - Assign that to
total
, replacing the current value.
- Add 1 to the current value of the loop variable
- We have to add
number + 1
becauserange
produces 0..9, not 1..10.
Classifying Errors
Is an indentation error a syntax error or a runtime error?
An IndentationError is a syntax error. Programs with syntax errors cannot be started. A program with a runtime error will start but an error will be thrown under certain conditions.
Line no | Variables |
---|---|
1 | total = 0 |
2 | total = 0 char = ‘t’ |
3 | total = 1 char = ‘t’ |
2 | total = 1 char = ‘i’ |
3 | total = 2 char = ‘i’ |
2 | total = 2 char = ‘n’ |
3 | total = 3 char = ‘n’ |
Practice Accumulating (continued)
Create an acronym: Starting from the list
["red", "green", "blue"]
, create the acronym
"RGB"
using a for loop.
Hint: You may need to use a string method to properly format the acronym.
Identifying Variable Name Errors
- Read the code below and try to identify what the errors are without running it.
- Run the code and read the error message. What type of
NameError
do you think this is? Is it a string with no quotes, a misspelled variable, or a variable that should have been defined but was not? - Fix the error.
- Repeat steps 2 and 3, until you have fixed all the errors.
- Python variable names are case sensitive:
number
andNumber
refer to different variables. - The variable
message
needs to be initialized as an empty string. - We want to add the string
"a"
tomessage
, not the undefined variablea
.
Ключові моменти
- A for loop executes commands once for each value in a collection.
- A
for
loop is made up of a collection, a loop variable, and a body. - The first line of the
for
loop must end with a colon, and the body must be indented. - Indentation is always meaningful in Python.
- Loop variables can be called anything (but it is strongly advised to have a meaningful name to the looping variable).
- The body of a loop can contain many statements.
- Use
range
to iterate over a sequence of numbers. - The Accumulator pattern turns many values into one.
Content from Conditionals
Останнє оновлення 2025-04-24 | Редагувати цю сторінку
Огляд
Питання
- How can programs do different things for different data?
Цілі
- Correctly write programs that use if and else statements and simple Boolean expressions (without logical operators).
- Trace the execution of unnested conditionals and conditionals inside loops.
Use if
statements to control whether or not a block of
code is executed.
- An
if
statement (more properly called a conditional statement) controls whether some block of code is executed or not. - Structure is similar to a
for
statement:- First line opens with
if
and ends with a colon - Body containing one or more statements is indented (usually by 4 spaces)
- First line opens with
PYTHON
mass = 3.54
if mass > 3.0:
print(mass, 'is large')
mass = 2.07
if mass > 3.0:
print (mass, 'is large')
ВИХІД
3.54 is large
Conditionals are often used inside loops.
- Not much point using a conditional when we know the value (as above).
- But useful when we have a collection to process.
ВИХІД
3.54 is large
9.22 is large
Use else
to execute a block of code when an
if
condition is not true.
-
else
can be used following anif
. - Allows us to specify an alternative to execute when the
if
branch isn’t taken.
PYTHON
masses = [3.54, 2.07, 9.22, 1.86, 1.71]
for m in masses:
if m > 3.0:
print(m, 'is large')
else:
print(m, 'is small')
ВИХІД
3.54 is large
2.07 is small
9.22 is large
1.86 is small
1.71 is small
Use elif
to specify additional tests.
- May want to provide several alternative choices, each with its own test.
- Use
elif
(short for “else if”) and a condition to specify these. - Always associated with an
if
. - Must come before the
else
(which is the “catch all”).
PYTHON
masses = [3.54, 2.07, 9.22, 1.86, 1.71]
for m in masses:
if m > 9.0:
print(m, 'is HUGE')
elif m > 3.0:
print(m, 'is large')
else:
print(m, 'is small')
ВИХІД
3.54 is large
2.07 is small
9.22 is HUGE
1.86 is small
1.71 is small
Conditions are tested once, in order.
- Python steps through the branches of the conditional in order, testing each in turn.
- So ordering matters.
PYTHON
grade = 85
if grade >= 90:
print('grade is A')
elif grade >= 80:
print('grade is B')
elif grade >= 70:
print('grade is C')
ВИХІД
grade is B
- Does not automatically go back and re-evaluate if values change.
PYTHON
velocity = 10.0
if velocity > 20.0:
print('moving too fast')
else:
print('adjusting velocity')
velocity = 50.0
ВИХІД
adjusting velocity
- Often use conditionals in a loop to “evolve” the values of variables.
PYTHON
velocity = 10.0
for i in range(5): # execute the loop 5 times
print(i, ':', velocity)
if velocity > 20.0:
print('moving too fast')
velocity = velocity - 5.0
else:
print('moving too slow')
velocity = velocity + 10.0
print('final velocity:', velocity)
ВИХІД
0 : 10.0
moving too slow
1 : 20.0
moving too slow
2 : 30.0
moving too fast
3 : 25.0
moving too fast
4 : 20.0
moving too slow
final velocity: 30.0
Create a table showing variables’ values to trace a program’s execution.
i | 0 | . | 1 | . | 2 | . | 3 | . | 4 | . |
velocity | 10.0 | 20.0 | . | 30.0 | . | 25.0 | . | 20.0 | . | 30.0 |
- The program must have a
print
statement outside the body of the loop to show the final value ofvelocity
, since its value is updated by the last iteration of the loop.
Compound Relations Using and
,
or
, and Parentheses
Often, you want some combination of things to be true. You can
combine relations within a conditional using and
and
or
. Continuing the example above, suppose you have
PYTHON
mass = [ 3.54, 2.07, 9.22, 1.86, 1.71]
velocity = [10.00, 20.00, 30.00, 25.00, 20.00]
i = 0
for i in range(5):
if mass[i] > 5 and velocity[i] > 20:
print("Fast heavy object. Duck!")
elif mass[i] > 2 and mass[i] <= 5 and velocity[i] <= 20:
print("Normal traffic")
elif mass[i] <= 2 and velocity[i] <= 20:
print("Slow light object. Ignore it")
else:
print("Whoa! Something is up with the data. Check it")
Just like with arithmetic, you can and should use parentheses
whenever there is possible ambiguity. A good general rule is to
always use parentheses when mixing and
and
or
in the same condition. That is, instead of:
write one of these:
PYTHON
if (mass[i] <= 2 or mass[i] >= 5) and velocity[i] > 20:
if mass[i] <= 2 or (mass[i] >= 5 and velocity[i] > 20):
so it is perfectly clear to a reader (and to Python) what you really mean.
ВИХІД
25.0
Trimming Values
Fill in the blanks so that this program creates a new list containing zeroes where the original list’s values were negative and ones where the original list’s values were positive.
PYTHON
original = [-1.5, 0.2, 0.4, 0.0, -1.3, 0.4]
result = ____
for value in original:
if ____:
result.append(0)
else:
____
print(result)
ВИХІД
[0, 1, 1, 1, 0, 1]
Initializing
Modify this program so that it finds the largest and smallest values in the list no matter what the range of values originally is.
PYTHON
values = [...some test data...]
smallest, largest = None, None
for v in values:
if ____:
smallest, largest = v, v
____:
smallest = min(____, v)
largest = max(____, v)
print(smallest, largest)
What are the advantages and disadvantages of using this method to find the range of the data?
PYTHON
values = [-2,1,65,78,-54,-24,100]
smallest, largest = None, None
for v in values:
if smallest is None and largest is None:
smallest, largest = v, v
else:
smallest = min(smallest, v)
largest = max(largest, v)
print(smallest, largest)
If you wrote == None
instead of is None
,
that works too, but Python programmers always write is None
because of the special way None
works in the language.
It can be argued that an advantage of using this method would be to
make the code more readable. However, a disadvantage is that this code
is not efficient because within each iteration of the for
loop statement, there are two more loops that run over two numbers each
(the min
and max
functions). It would be more
efficient to iterate over each number just once:
PYTHON
values = [-2,1,65,78,-54,-24,100]
smallest, largest = None, None
for v in values:
if smallest is None or v < smallest:
smallest = v
if largest is None or v > largest:
largest = v
print(smallest, largest)
Now we have one loop, but four comparison tests. There are two ways we could improve it further: either use fewer comparisons in each iteration, or use two loops that each contain only one comparison test. The simplest solution is often the best:
Ключові моменти
- Use
if
statements to control whether or not a block of code is executed. - Conditionals are often used inside loops.
- Use
else
to execute a block of code when anif
condition is not true. - Use
elif
to specify additional tests. - Conditions are tested once, in order.
- Create a table showing variables’ values to trace a program’s execution.
Content from Looping Over Data Sets
Останнє оновлення 2025-01-24 | Редагувати цю сторінку
Огляд
Питання
- How can I process many data sets with a single command?
Цілі
- Be able to read and write globbing expressions that match sets of files.
- Use glob to create lists of files.
- Write for loops to perform operations on files given their names in a list.
Use a for
loop to process files given a list of their
names.
- A filename is a character string.
- And lists can contain character strings.
PYTHON
import pandas as pd
for filename in ['data/gapminder_gdp_africa.csv', 'data/gapminder_gdp_asia.csv']:
data = pd.read_csv(filename, index_col='country')
print(filename, data.min())
ВИХІД
data/gapminder_gdp_africa.csv gdpPercap_1952 298.846212
gdpPercap_1957 335.997115
gdpPercap_1962 355.203227
gdpPercap_1967 412.977514
⋮ ⋮ ⋮
gdpPercap_1997 312.188423
gdpPercap_2002 241.165877
gdpPercap_2007 277.551859
dtype: float64
data/gapminder_gdp_asia.csv gdpPercap_1952 331
gdpPercap_1957 350
gdpPercap_1962 388
gdpPercap_1967 349
⋮ ⋮ ⋮
gdpPercap_1997 415
gdpPercap_2002 611
gdpPercap_2007 944
dtype: float64
Use glob.glob
to find sets of files whose names match a pattern.
- In Unix, the term “globbing” means “matching a set of files with a pattern”.
- The most common patterns are:
-
*
meaning “match zero or more characters” -
?
meaning “match exactly one character”
-
- Python’s standard library contains the
glob
module to provide pattern matching functionality - The
glob
module contains a function also calledglob
to match file patterns - E.g.,
glob.glob('*.txt')
matches all files in the current directory whose names end with.txt
. - Result is a (possibly empty) list of character strings.
ВИХІД
all csv files in data directory: ['data/gapminder_all.csv', 'data/gapminder_gdp_africa.csv', \
'data/gapminder_gdp_americas.csv', 'data/gapminder_gdp_asia.csv', 'data/gapminder_gdp_europe.csv', \
'data/gapminder_gdp_oceania.csv']
ВИХІД
all PDB files: []
Use glob
and for
to process batches of
files.
- Helps a lot if the files are named and stored systematically and consistently so that simple patterns will find the right data.
PYTHON
for filename in glob.glob('data/gapminder_*.csv'):
data = pd.read_csv(filename)
print(filename, data['gdpPercap_1952'].min())
ВИХІД
data/gapminder_all.csv 298.8462121
data/gapminder_gdp_africa.csv 298.8462121
data/gapminder_gdp_americas.csv 1397.717137
data/gapminder_gdp_asia.csv 331.0
data/gapminder_gdp_europe.csv 973.5331948
data/gapminder_gdp_oceania.csv 10039.59564
- This includes all data, as well as per-region data.
- Use a more specific pattern in the exercises to exclude the whole data set.
- But note that the minimum of the entire data set is also the minimum of one of the data sets, which is a nice check on correctness.
Determining Matches
Which of these files is not matched by the expression
glob.glob('data/*as*.csv')
?
data/gapminder_gdp_africa.csv
data/gapminder_gdp_americas.csv
data/gapminder_gdp_asia.csv
1 is not matched by the glob.
Minimum File Size
Modify this program so that it prints the number of records in the file that has the fewest records.
PYTHON
import glob
import pandas as pd
fewest = ____
for filename in glob.glob('data/*.csv'):
dataframe = pd.____(filename)
fewest = min(____, dataframe.shape[0])
print('smallest file has', fewest, 'records')
Note that the DataFrame.shape()
method returns a tuple with the number of rows and columns of the
data frame.
PYTHON
import glob
import pandas as pd
fewest = float('Inf')
for filename in glob.glob('data/*.csv'):
dataframe = pd.read_csv(filename)
fewest = min(fewest, dataframe.shape[0])
print('smallest file has', fewest, 'records')
You might have chosen to initialize the fewest
variable
with a number greater than the numbers you’re dealing with, but that
could lead to trouble if you reuse the code with bigger numbers. Python
lets you use positive infinity, which will work no matter how big your
numbers are. What other special strings does the float
function recognize?
Comparing Data
Write a program that reads in the regional data sets and plots the average GDP per capita for each region over time in a single chart. Pandas will raise an error if it encounters non-numeric columns in a dataframe computation so you may need to either filter out those columns or tell pandas to ignore them.
This solution builds a useful legend by using the string
split
method to extract the region
from
the path ’data/gapminder\_gdp\_a\_specific\_region.csv’.
PYTHON
import glob
import pandas as pd
import matplotlib.pyplot as plt
fig, ax = plt.subplots(1,1)
for filename in glob.glob('data/gapminder_gdp*.csv'):
dataframe = pd.read_csv(filename)
# extract <region> from the filename, expected to be in the format 'data/gapminder_gdp_<region>.csv'.
# we will split the string using the split method and `_` as our separator,
# retrieve the last string in the list that split returns (`<region>.csv`),
# and then remove the `.csv` extension from that string.
# NOTE: the pathlib module covered in the next callout also offers
# convenient abstractions for working with filesystem paths and could solve this as well:
# from pathlib import Path
# region = Path(filename).stem.split('_')[-1]
region = filename.split('_')[-1][:-4]
# extract the years from the columns of the dataframe
headings = dataframe.columns[1:]
years = headings.str.split('_').str.get(1)
# pandas raises errors when it encounters non-numeric columns in a dataframe computation
# but we can tell pandas to ignore them with the `numeric_only` parameter
dataframe.mean(numeric_only=True).plot(ax=ax, label=region)
# NOTE: another way of doing this selects just the columns with gdp in their name using the filter method
# dataframe.filter(like="gdp").mean().plot(ax=ax, label=region)
# set the title and labels
ax.set_title('GDP Per Capita for Regions Over Time')
ax.set_xticks(range(len(years)))
ax.set_xticklabels(years)
ax.set_xlabel('Year')
plt.tight_layout()
plt.legend()
plt.show()
Dealing with File Paths
The pathlib
module provides useful abstractions for file and path manipulation
like returning the name of a file without the file extension. This is
very useful when looping over files and directories. In the example
below, we create a Path
object and inspect its
attributes.
PYTHON
from pathlib import Path
p = Path("data/gapminder_gdp_africa.csv")
print(p.parent)
print(p.stem)
print(p.suffix)
ВИХІД
data
gapminder_gdp_africa
.csv
Hint: Check all available attributes and methods on
the Path
object with the dir()
function.
Ключові моменти
- Use a
for
loop to process files given a list of their names. - Use
glob.glob
to find sets of files whose names match a pattern. - Use
glob
andfor
to process batches of files.
Content from Післяобідня кава
Останнє оновлення 2025-02-23 | Редагувати цю сторінку
Reflection exercise
Over break, reflect on and discuss the following:
- A common refrain in software engineering is “Don’t Repeat Yourself”. How do the techniques we’ve learned in the last lessons help us avoid repeating ourselves? Note that in practice there is some nuance to this and should be balanced with doing the simplest thing that could possibly work.
- What are the pros / cons of making a variable global or local to a function?
- When would you consider turning a block of code into a function definition?
Content from Writing Functions
Останнє оновлення 2024-10-08 | Редагувати цю сторінку
Огляд
Питання
- How can I create my own functions?
Цілі
- Explain and identify the difference between function definition and function call.
- Write a function that takes a small, fixed number of arguments and produces a single result.
Break programs down into functions to make them easier to understand.
- Human beings can only keep a few items in working memory at a time.
- Understand larger/more complicated ideas by understanding and
combining pieces.
- Components in a machine.
- Lemmas when proving theorems.
- Functions serve the same purpose in programs.
- Encapsulate complexity so that we can treat it as a single “thing”.
- Also enables re-use.
- Write one time, use many times.
Define a function using def
with a name, parameters,
and a block of code.
- Begin the definition of a new function with
def
. - Followed by the name of the function.
- Must obey the same rules as variable names.
- Then parameters in parentheses.
- Empty parentheses if the function doesn’t take any inputs.
- We will discuss this in detail in a moment.
- Then a colon.
- Then an indented block of code.
Defining a function does not run it.
- Defining a function does not run it.
- Like assigning a value to a variable.
- Must call the function to execute the code it contains.
ВИХІД
Hello!
Arguments in a function call are matched to its defined parameters.
- Functions are most useful when they can operate on different data.
- Specify parameters when defining a function.
- These become variables when the function is executed.
- Are assigned the arguments in the call (i.e., the values passed to the function).
- If you don’t name the arguments when using them in the call, the arguments will be matched to parameters in the order the parameters are defined in the function.
PYTHON
def print_date(year, month, day):
joined = str(year) + '/' + str(month) + '/' + str(day)
print(joined)
print_date(1871, 3, 19)
ВИХІД
1871/3/19
Or, we can name the arguments when we call the function, which allows us to specify them in any order and adds clarity to the call site; otherwise as one is reading the code they might forget if the second argument is the month or the day for example.
ВИХІД
1871/3/19
- Via Twitter:
()
contains the ingredients for the function while the body contains the recipe.
Functions may return a result to their caller using
return
.
- Use
return ...
to give a value back to the caller. - May occur anywhere in the function.
- But functions are easier to understand if
return
occurs:- At the start to handle special cases.
- At the very end, with a final result.
ВИХІД
average of actual values: 2.6666666666666665
ВИХІД
average of empty list: None
- Remember: every function returns something.
- A function that doesn’t explicitly
return
a value automatically returnsNone
.
ВИХІД
1871/3/19
result of call is: None
Identifying Syntax Errors
- Read the code below and try to identify what the errors are without running it.
- Run the code and read the error message. Is it a
SyntaxError
or anIndentationError
? - Fix the error.
- Repeat steps 2 and 3 until you have fixed all the errors.
ВИХІД
calling <function report at 0x7fd128ff1bf8> 22.5
A function call always needs parenthesis, otherwise you get memory address of the function object. So, if we wanted to call the function named report, and give it the value 22.5 to report on, we could have our function call as follows
ВИХІД
calling
pressure is 22.5
Order of Operations
- What’s wrong in this example?
PYTHON
result = print_time(11, 37, 59)
def print_time(hour, minute, second):
time_string = str(hour) + ':' + str(minute) + ':' + str(second)
print(time_string)
- After fixing the problem above, explain why running this example code:
gives this output:
ВИХІД
11:37:59
result of call is: None
- Why is the result of the call
None
?
The problem with the example is that the function
print_time()
is defined after the call to the function is made. Python doesn’t know how to resolve the nameprint_time
since it hasn’t been defined yet and will raise aNameError
e.g.,NameError: name 'print_time' is not defined
The first line of output
11:37:59
is printed by the first line of code,result = print_time(11, 37, 59)
that binds the value returned by invokingprint_time
to the variableresult
. The second line is from the second print call to print the contents of theresult
variable.print_time()
does not explicitlyreturn
a value, so it automatically returnsNone
.
Find the First
Calling by Name
Earlier we saw this function:
PYTHON
def print_date(year, month, day):
joined = str(year) + '/' + str(month) + '/' + str(day)
print(joined)
We saw that we can call the function using named arguments, like this:
- What does
print_date(day=1, month=2, year=2003)
print? - When have you seen a function call like this before?
- When and why is it useful to call functions this way?
2003/2/1
We saw examples of using named arguments when working with the pandas library. For example, when reading in a dataset using
data = pd.read_csv('data/gapminder_gdp_europe.csv', index_col='country')
, the last argumentindex_col
is a named argument.Using named arguments can make code more readable since one can see from the function call what name the different arguments have inside the function. It can also reduce the chances of passing arguments in the wrong order, since by using named arguments the order doesn’t matter.
Encapsulation of an If/Print Block
The code below will run on a label-printer for chicken eggs. A digital scale will report a chicken egg mass (in grams) to the computer and then the computer will print a label.
PYTHON
import random
for i in range(10):
# simulating the mass of a chicken egg
# the (random) mass will be 70 +/- 20 grams
mass = 70 + 20.0 * (2.0 * random.random() - 1.0)
print(mass)
# egg sizing machinery prints a label
if mass >= 85:
print("jumbo")
elif mass >= 70:
print("large")
elif mass < 70 and mass >= 55:
print("medium")
else:
print("small")
The if-block that classifies the eggs might be useful in other
situations, so to avoid repeating it, we could fold it into a function,
get_egg_label()
. Revising the program to use the function
would give us this:
PYTHON
# revised version
import random
for i in range(10):
# simulating the mass of a chicken egg
# the (random) mass will be 70 +/- 20 grams
mass = 70 + 20.0 * (2.0 * random.random() - 1.0)
print(mass, get_egg_label(mass))
- Create a function definition for
get_egg_label()
that will work with the revised program above. Note that theget_egg_label()
function’s return value will be important. Sample output from the above program would be71.23 large
. - A dirty egg might have a mass of more than 90 grams, and a spoiled
or broken egg will probably have a mass that’s less than 50 grams.
Modify your
get_egg_label()
function to account for these error conditions. Sample output could be25 too light, probably spoiled
.
PYTHON
def get_egg_label(mass):
# egg sizing machinery prints a label
egg_label = "Unlabelled"
if mass >= 90:
egg_label = "warning: egg might be dirty"
elif mass >= 85:
egg_label = "jumbo"
elif mass >= 70:
egg_label = "large"
elif mass < 70 and mass >= 55:
egg_label = "medium"
elif mass < 50:
egg_label = "too light, probably spoiled"
else:
egg_label = "small"
return egg_label
Encapsulating Data Analysis
Assume that the following code has been executed:
PYTHON
import pandas as pd
data_asia = pd.read_csv('data/gapminder_gdp_asia.csv', index_col=0)
japan = data_asia.loc['Japan']
- Complete the statements below to obtain the average GDP for Japan across the years reported for the 1980s.
PYTHON
year = 1983
gdp_decade = 'gdpPercap_' + str(year // ____)
avg = (japan.loc[gdp_decade + ___] + japan.loc[gdp_decade + ___]) / 2
- Abstract the code above into a single function.
PYTHON
def avg_gdp_in_decade(country, continent, year):
data_countries = pd.read_csv('data/gapminder_gdp_'+___+'.csv',delimiter=',',index_col=0)
____
____
____
return avg
- How would you generalize this function if you did not know beforehand which specific years occurred as columns in the data? For instance, what if we also had data from years ending in 1 and 9 for each decade? (Hint: use the columns to filter out the ones that correspond to the decade, instead of enumerating them in the code.)
- The average GDP for Japan across the years reported for the 1980s is computed with:
PYTHON
year = 1983
gdp_decade = 'gdpPercap_' + str(year // 10)
avg = (japan.loc[gdp_decade + '2'] + japan.loc[gdp_decade + '7']) / 2
- That code as a function is:
PYTHON
def avg_gdp_in_decade(country, continent, year):
data_countries = pd.read_csv('data/gapminder_gdp_' + continent + '.csv', index_col=0)
c = data_countries.loc[country]
gdp_decade = 'gdpPercap_' + str(year // 10)
avg = (c.loc[gdp_decade + '2'] + c.loc[gdp_decade + '7'])/2
return avg
- To obtain the average for the relevant years, we need to loop over them:
PYTHON
def avg_gdp_in_decade(country, continent, year):
data_countries = pd.read_csv('data/gapminder_gdp_' + continent + '.csv', index_col=0)
c = data_countries.loc[country]
gdp_decade = 'gdpPercap_' + str(year // 10)
total = 0.0
num_years = 0
for yr_header in c.index: # c's index contains reported years
if yr_header.startswith(gdp_decade):
total = total + c.loc[yr_header]
num_years = num_years + 1
return total/num_years
The function can now be called by:
ВИХІД
20880.023800000003
Simulating a dynamical system
In mathematics, a dynamical system is a system in which a function describes the time dependence of a point in a geometrical space. A canonical example of a dynamical system is the logistic map, a growth model that computes a new population density (between 0 and 1) based on the current density. In the model, time takes discrete values 0, 1, 2, …
- Define a function called
logistic_map
that takes two inputs:x
, representing the current population (at timet
), and a parameterr = 1
. This function should return a value representing the state of the system (population) at timet + 1
, using the mapping function:
f(t+1) = r * f(t) * [1 - f(t)]
Using a
for
orwhile
loop, iterate thelogistic_map
function defined in part 1, starting from an initial population of 0.5, for a period of timet_final = 10
. Store the intermediate results in a list so that after the loop terminates you have accumulated a sequence of values representing the state of the logistic map at timest = [0,1,...,t_final]
(11 values in total). Print this list to see the evolution of the population.Encapsulate the logic of your loop into a function called
iterate
that takes the initial population as its first input, the parametert_final
as its second input and the parameterr
as its third input. The function should return the list of values representing the state of the logistic map at timest = [0,1,...,t_final]
. Run this function for periodst_final = 100
and1000
and print some of the values. Is the population trending toward a steady state?
PYTHON
initial_population = 0.5
t_final = 10
r = 1.0
population = [initial_population]
for t in range(t_final):
population.append( logistic_map(population[t], r) )
PYTHON
def iterate(initial_population, t_final, r):
population = [initial_population]
for t in range(t_final):
population.append( logistic_map(population[t], r) )
return population
for period in (10, 100, 1000):
population = iterate(0.5, period, 1)
print(population[-1])
ВИХІД
0.06945089389714401
0.009395779870614648
0.0009913908614406382
The population seems to be approaching zero.
Using Functions With Conditionals in Pandas
Functions will often contain conditionals. Here is a short example that will indicate which quartile the argument is in based on hand-coded values for the quartile cut points.
PYTHON
def calculate_life_quartile(exp):
if exp < 58.41:
# This observation is in the first quartile
return 1
elif exp >= 58.41 and exp < 67.05:
# This observation is in the second quartile
return 2
elif exp >= 67.05 and exp < 71.70:
# This observation is in the third quartile
return 3
elif exp >= 71.70:
# This observation is in the fourth quartile
return 4
else:
# This observation has bad data
return None
calculate_life_quartile(62.5)
ВИХІД
2
That function would typically be used within a for
loop,
but Pandas has a different, more efficient way of doing the same thing,
and that is by applying a function to a dataframe or a portion
of a dataframe. Here is an example, using the definition above.
PYTHON
data = pd.read_csv('data/gapminder_all.csv')
data['life_qrtl'] = data['lifeExp_1952'].apply(calculate_life_quartile)
There is a lot in that second line, so let’s take it piece by piece.
On the right side of the =
we start with
data['lifeExp']
, which is the column in the dataframe
called data
labeled lifExp
. We use the
apply()
to do what it says, apply the
calculate_life_quartile
to the value of this column for
every row in the dataframe.
Ключові моменти
- Break programs down into functions to make them easier to understand.
- Define a function using
def
with a name, parameters, and a block of code. - Defining a function does not run it.
- Arguments in a function call are matched to its defined parameters.
- Functions may return a result to their caller using
return
.
Content from Variable Scope
Останнє оновлення 2024-07-02 | Редагувати цю сторінку
Огляд
Питання
- How do function calls actually work?
- How can I determine where errors occurred?
Цілі
- Identify local and global variables.
- Identify parameters as local variables.
- Read a traceback and determine the file, function, and line number on which the error occurred, the type of error, and the error message.
The scope of a variable is the part of a program that can ‘see’ that variable.
- There are only so many sensible names for variables.
- People using functions shouldn’t have to worry about what variable names the author of the function used.
- People writing functions shouldn’t have to worry about what variable names the function’s caller uses.
- The part of a program in which a variable is visible is called its scope.
-
pressure
is a global variable.- Defined outside any particular function.
- Visible everywhere.
-
t
andtemperature
are local variables inadjust
.- Defined in the function.
- Not visible in the main program.
- Remember: a function parameter is a variable that is automatically assigned a value when the function is called.
ВИХІД
adjusted: 0.01238691049085659
ПОМИЛКА
Traceback (most recent call last):
File "/Users/swcarpentry/foo.py", line 8, in <module>
print('temperature after call:', temperature)
NameError: name 'temperature' is not defined
Reading Error Messages
Read the traceback below, and identify the following:
- How many levels does the traceback have?
- What is the file name where the error occurred?
- What is the function name where the error occurred?
- On which line number in this function did the error occur?
- What is the type of error?
- What is the error message?
ПОМИЛКА
---------------------------------------------------------------------------
KeyError Traceback (most recent call last)
<ipython-input-2-e4c4cbafeeb5> in <module>()
1 import errors_02
----> 2 errors_02.print_friday_message()
/Users/ghopper/thesis/code/errors_02.py in print_friday_message()
13
14 def print_friday_message():
---> 15 print_message("Friday")
/Users/ghopper/thesis/code/errors_02.py in print_message(day)
9 "sunday": "Aw, the weekend is almost over."
10 }
---> 11 print(messages[day])
12
13
KeyError: 'Friday'
Three levels.
errors_02.py
print_message
Line 11
KeyError
. These errors occur when we are trying to look up a key that does not exist (usually in a data structure such as a dictionary). We can find more information about theKeyError
and other built-in exceptions in the Python docs.KeyError: 'Friday'
Ключові моменти
- The scope of a variable is the part of a program that can ‘see’ that variable.
Content from Стиль програмування
Останнє оновлення 2024-07-26 | Редагувати цю сторінку
Огляд
Питання
- Як я можу зробити свої програми більш читабельними?
- Як більшість програмістів форматують свій код?
- How can programs check their own operation?
Цілі
- Дотримуйтесь основних правил стилю кодування.
- Виконуйте рефакторинг односторінкових програм, щоб зробити їх більш читабельними та обґрунтувати зміни.
- Use Python community coding standards (PEP-8).
Coding style
A consistent coding style helps others (including our future selves) read and understand code more easily. Code is read much more often than it is written, and as the Zen of Python states, “Readability counts”. Python proposed a standard style through one of its first Python Enhancement Proposals (PEP), PEP8.
Some points worth highlighting:
- document your code and ensure that assumptions, internal algorithms, expected inputs, expected outputs, etc., are clear
- use clear, semantically meaningful variable names
- use white-space, not tabs, to indent lines (tabs can cause problems across different text editors, operating systems, and version control systems)
Follow standard Python style in your code.
-
PEP8: a style
guide for Python that discusses topics such as how to name variables,
how to indent your code, how to structure your
import
statements, etc. Adhering to PEP8 makes it easier for other Python developers to read and understand your code, and to understand what their contributions should look like. - To check your code for compliance with PEP8, you can use the pycodestyle application and tools like the black code formatter can automatically format your code to conform to PEP8 and pycodestyle (a Jupyter notebook formatter also exists nb_black).
- Some groups and organizations follow different style guidelines besides PEP8. For example, the Google style guide on Python makes slightly different recommendations. Google wrote an application that can help you format your code in either their style or PEP8 called yapf.
- With respect to coding style, the key is consistency. Choose a style for your project be it PEP8, the Google style, or something else and do your best to ensure that you and anyone else you are collaborating with sticks to it. Consistency within a project is often more impactful than the particular style used. A consistent style will make your software easier to read and understand for others and for your future self.
Use assertions to check for internal errors.
Assertions are a simple but powerful method for making sure that the context in which your code is executing is as you expect.
PYTHON
def calc_bulk_density(mass, volume):
'''Return dry bulk density = powder mass / powder volume.'''
assert volume > 0
return mass / volume
If the assertion is False
, the Python interpreter raises
an AssertionError
runtime exception. The source code for
the expression that failed will be displayed as part of the error
message. To ignore assertions in your code run the interpreter with the
‘-O’ (optimize) switch. Assertions should contain only simple checks and
never change the state of the program. For example, an assertion should
never contain an assignment.
Use docstrings to provide builtin help.
If the first thing in a function is a character string that is not assigned directly to a variable, Python attaches it to the function, accessible via the builtin help function. This string that provides documentation is also known as a docstring.
PYTHON
def average(values):
"Return average of values, or None if no values are supplied."
if len(values) == 0:
return None
return sum(values) / len(values)
help(average)
ВИХІД
Help on function average in module __main__:
average(values)
Return average of values, or None if no values are supplied.
What Will Be Shown?
Highlight the lines in the code below that will be available as online help. Are there lines that should be made available, but won’t be? Will any lines produce a syntax error or a runtime error?
PYTHON
"Find maximum edit distance between multiple sequences."
# This finds the maximum distance between all sequences.
def overall_max(sequences):
'''Determine overall maximum edit distance.'''
highest = 0
for left in sequences:
for right in sequences:
'''Avoid checking sequence against itself.'''
if left != right:
this = edit_distance(left, right)
highest = max(highest, this)
# Report.
return highest
Document This
Use comments to describe and help others understand potentially unintuitive sections or individual lines of code. They are especially useful to whoever may need to understand and edit your code in the future, including yourself.
Use docstrings to document the acceptable inputs and expected outputs
of a method or class, its purpose, assumptions and intended behavior.
Docstrings are displayed when a user invokes the builtin
help
method on your method or class.
Turn the comment in the following function into a docstring and check
that help
displays it properly.
Clean Up This Code
- Read this short program and try to predict what it does.
- Run it: how accurate was your prediction?
- Refactor the program to make it more readable. Remember to run it after each change to ensure its behavior hasn’t changed.
- Compare your rewrite with your neighbor’s. What did you do the same? What did you do differently, and why?
Here’s one solution.
PYTHON
def string_machine(input_string, iterations):
"""
Takes input_string and generates a new string with -'s and *'s
corresponding to characters that have identical adjacent characters
or not, respectively. Iterates through this procedure with the resultant
strings for the supplied number of iterations.
"""
print(input_string)
input_string_length = len(input_string)
old = input_string
for i in range(iterations):
new = ''
# iterate through characters in previous string
for j in range(input_string_length):
left = j-1
right = (j+1) % input_string_length # ensure right index wraps around
if old[left] == old[right]:
new = new + '-'
else:
new = new + '*'
print(new)
# store new string as old
old = new
string_machine('et cetera', 10)
ВИХІД
et cetera
*****-***
----*-*--
---*---*-
--*-*-*-*
**-------
***-----*
--**---**
*****-***
----*-*--
---*---*-
Ключові моменти
- Follow standard Python style in your code.
- Use docstrings to provide builtin help.
Content from Wrap-Up
Останнє оновлення 2024-07-02 | Редагувати цю сторінку
Огляд
Питання
- What have we learned?
- What else is out there and where do I find it?
Цілі
- Name and locate scientific Python community sites for software, workshops, and help.
Leslie Lamport once said, “Writing is nature’s way of showing you how sloppy your thinking is.” The same is true of programming: many things that seem obvious when we’re thinking about them turn out to be anything but when we have to explain them precisely.
Python supports a large and diverse community across academia and industry.
The Python 3 documentation covers the core language and the standard library.
PyCon is the largest annual conference for the Python community.
SciPy is a rich collection of scientific utilities. It is also the name of a series of annual conferences.
Jupyter is the home of Project Jupyter.
Pandas is the home of the Pandas data library.
Stack Overflow’s general Python section can be helpful, as well as the sections on NumPy, SciPy, and Pandas.
Ключові моменти
- Python supports a large and diverse community across academia and industry.
Content from Feedback
Останнє оновлення 2024-07-02 | Редагувати цю сторінку
Огляд
Питання
- How did the class go?
Цілі
- Gather feedback on the class
Gather feedback from participants.
Ключові моменти
- We are constantly seeking to improve this course.